D&D4 - Nanoporous Materials
Group Leaders
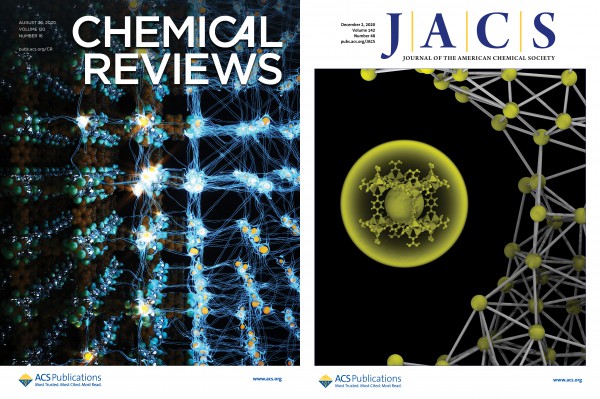
Left: MOFs are made for big-data science: The neurons of artificial neural networks, representing the big-data approach to chemistry, become one with the framework of a metal-organic framework (figure by Alexander Tokarev, from [1]).
Right: one out of a million; each data point represents a metal-organic framework used to discover novel materials for carbon capture (figure from [2]).
[1] K. M. Jablonka, D. Ongari, S. M. Moosavi, and B. Smit, Big-Data Science in Porous Materials: Materials Genomics and Machine Learning, Chemical Reviews 120, 8066 (2020). [Open Access URL]
[2] S. M. Moosavi, K. M. Jablonka, and B. Smit, The Role of Machine Learning in the Understanding and Design of Materials, Journal of the American Chemical Society 142, 20273 (2020). [Open Access URL]
Related publications (until January 2023)
- S. Falletta, A. Pasquarello, Polarons free from many-body self-interaction in density functional theory, Physical Review B 106, 125119 (2022). [Open Access URL]
Dataset on Materials Cloud.
Group(s): Pasquarello / Project(s): DD4 - S. Falletta, A. Pasquarello, Many-Body Self-Interaction and Polarons, Physical Review Letters 129, 126401 (2022). [Open Access URL]
Dataset on Materials Cloud.
Group(s): Pasquarello / Project(s): DD4 - G. Patrick, D. Cartagenova, M. Ranocchiari, Phosphine-Functionalized Porous Materials for Catalytic Organic Synthesis, Chemistry–A European Journal 2022, e202201006 (2022). [Open Access URL]
Group(s): Ranocchiari / Project(s): DD4 - A. Hehn, B. Sertcan, F. Belleflamme, S. K. Chulkov, M. B. Watkins, J. Hutter, Excited-state properties for extended systems: Efficient hybrid density functional methods., Journal of Chemical Theory and Computation 18, 4186–4202 (2022). [Open Access URL]
Dataset on Materials Cloud.
Group(s): Hutter / Project(s): DD4 - D. Hua, J. Huang, E. Fabbri, M. Rafique, B. Song, Development of Anion Exchange Membrane Water Electrolysis and the Associated Challenges: A Review, ChemElectroChem 10 (2023). [Open Access URL]
Group(s): Fabbri / Project(s): DD4 - J. Yang, S. Falletta, A. Pasquarello, One-Shot Approach for Enforcing Piecewise Linearity on Hybrid Functionals: Application to Band Gap Predictions, The Journal of Physical Chemistry Letters 13, 3066–3071 (2022). [Open Access URL]
Dataset on Materials Cloud.
Group(s): Pasquarello / Project(s): DD4
- A. Bussy, J. Hutter, First-principles correction scheme for linear-response time-dependent density functional theory calculations of core electronic states, The Journal of Chemical Physics 155, 034108 (2021). [Open Access URL]
Dataset on Materials Cloud.
Group(s): Hutter / Project(s): DD4 - J. M. d. Almeida, N. L. Nguyen, N. Colonna, W. Chen, C. R. Miranda, A. Pasquarello, N. Marzari, Electronic Structure of Water from Koopmans-Compliant Functionals, Journal of Chemical Theory and Computation 17, 3923–3930 (2021). [Open Access URL]
Dataset on Materials Cloud.
Group(s): Marzari, Pasquarello / Project(s): DD4, OSP - Y. S. Al-Hamdani, P. R. Nagy, A. Zen, D. Barton, M. Kállay, J. G. Brandenburg, A. Tkatchenko, Interactions between large molecules pose a puzzle for reference quantum mechanical methods, Nature Communications 12, 3927 (2021). [Open Access URL]
Group(s): Hutter / Project(s): DD4 - J. Wilhelm, P. Seewald, D. Golze, Low-Scaling GW with Benchmark Accuracy and Application to Phosphorene Nanosheets, Journal of Chemical Theory and Computation 17, 1662–1677 (2021). [Open Access URL]
Group(s): Hutter / Project(s): DD4 - J. Lan, V. Kapil, P. Gasparotto, M. Ceriotti, M. Iannuzzi, V. V. Rybkin, Simulating the ghost: quantum dynamics of the solvated electron, Nature Communications 12, 766 (2021). [Open Access URL]
Dataset on Materials Cloud.
Group(s): Ceriotti, Hutter / Project(s): DD1, DD4 - J. Linke, T. Rohrbach, M. Ranocchiari, T. J. Schmidt, E. Fabbri, Enlightening the journey of metal-organic framework (derived) catalysts during the oxygen evolution reaction in alkaline media via operando X-ray absorption spectroscopy, Current Opinion in Electrochemistry 30, 100845 (2021). [Open Access URL]
Group(s): Fabbri, Ranocchiari / Project(s): DD4 - A. Bussy, J. Hutter, Efficient and low-scaling linear-response time-dependent density functional theory implementation for core-level spectroscopy of large and periodic systems, Physical Chemistry Chemical Physics 23, 4736–4746 (2021). [Open Access URL]
Dataset on Materials Cloud.
Group(s): Hutter / Project(s): DD4 - L. Sévery, J. Szczerbiński, M. Taskin, I. Tuncay, F. Brandalise Nunes, C. Cignarella, G. Tocci, O. Blacque, J. Osterwalder, R. Zenobi, M. Iannuzzi, S. D. Tilley, Immobilization of molecular catalysts on electrode surfaces using host-guest interactions, Nature Chemistry 13, 523–529 (2021). [Open Access URL]
Dataset on DataDryad.
Group(s): Hutter / Project(s): DD4 - R. Kobayashi, T. P. M. Goumans, N. O. Carstensen, T. M. Soini, N. Marzari, I. Timrov, S. Poncé, E. B. Linscott, C. J. Sewell, G. Pizzi, F. Ramirez, M. Bercx, S. P. Huber, C. S. Adorf, L. Talirz, Virtual Computational Chemistry Teaching Laboratories-Hands-On at a Distance, Journal of Chemical Education 98, 3163–3171 (2021). [Open Access URL]
Group(s): Marzari, Pizzi / Project(s): DD4, OSP - K. M. Jablonka, M. ë. Zasso, L. Patiny, N. Marzari, G. Pizzi, B. Smit, A. V. Yakutovich, Connecting lab experiments with computer experiments: Making “routine” simulations routine, ChemRxiv. Preprint. (2021). [Open Access URL]
Group(s): Marzari, Pizzi, Smit / Project(s): DD4, OSP - S. P. Huber, E. Bosoni, M. Bercx, J. Bröder, A. Degomme, V. Dikan, K. Eimre, E. Flage-Larsen, A. Garcia, L. Genovese, D. Gresch, C. Johnston, G. Petretto, S. Poncé, G. Rignanese, C. J. Sewell, B. Smit, V. Tseplyaev, M. Uhrin, D. Wortmann, A. V. Yakutovich, A. Zadoks, P. Zarabadi-Poor, B. Zhu, N. Marzari, G. Pizzi, Common workflows for computing material properties using different quantum engines, npj Computational Materials 7, 136 (2021). [Open Access URL]
Dataset on Materials Cloud.
Group(s): Marzari, Passerone, Pizzi, Smit / Project(s): DD3, DD4, OSP - K. M. Jablonka, G. M. Jothiappan, S. Wang, B. Smit, B. Yoo, Bias free multiobjective active learning for materials design and discovery, Nature Communications 12, 2312 (2021). [Open Access URL]
Dataset on Materials Cloud.
Group(s): Smit / Project(s): DD4 - K. M. Jablonka, D. Ongari, S. M. Moosavi, B. Smit, Using collective knowledge to assign oxidation states of metal cations in metal-organic frameworks, Nature Chemistry 13, 771 (2021). [Open Access URL]
Dataset on Materials Cloud.
Group(s): Smit / Project(s): DD4 - F. P. Kinik, A. Ortega-Guerrero, D. Ongari, C. P. Ireland, B. Smit, Pyrene-based metal organic frameworks: from synthesis to applications, Chemical Society Reviews 50, 3143–3177 (2021). [Open Access URL]
Dataset on Materials Cloud.
Group(s): Smit / Project(s): DD4 - L. Talirz, L. M. Ghiringhelli, B. Smit, Trends in Atomistic Simulation Software Usage [Article v1.0], Living Journal of Computational Molecular Science 3, 1483 (2021). [Open Access URL]
Dataset .
Group(s): Marzari, Pizzi, Smit / Project(s): DD4, OSP - K. M. Jablonka, S. M. Moosavi, M. Asgari, C. Ireland, L. Patiny, B. Smit, A data-driven perspective on the colours of metal-organic frameworks, Chemical Science 12, 3587–3598 (2021). [Open Access URL]
Dataset on Materials Cloud.
Group(s): Smit / Project(s): DD4 - A. V. Yakutovich, O. S. Kristjan Eimre, L. Talirz, C. S. Adorf, C. W. Andersen, E. Ditler, D. Du, D. Passerone, B. Smit, N. Marzari, G. Pizzi, C. A. Pignedoli, AiiDAlab – an ecosystem for developing, executing, and sharing scientific workflows, Computational Materials Science 188, 110165 (2021). [Open Access URL]
Dataset on GitHub.
Group(s): Marzari, Passerone, Pizzi, Smit / Project(s): DD4, OSP - D. Ongari, L. Talirz, B. Smit, Too Many Materials and Too Many Applications: An Experimental Problem Waiting for a Computational Solution, ACS Central Science 6, 1898 (2020). [Open Access URL]
Dataset on Materials Cloud.
Group(s): Smit / Project(s): DD4, OSP - M. Fumanal, A. Ortega-Guerrero, K. M. Jablonka, B. Smit, I. Tavernelli, Charge Separation and Charge Carrier Mobility in Photocatalytic Metal-Organic Frameworks, Advanced Functional Materials 30, 2003792 (2020). [Open Access URL]
Dataset on Materials Cloud.
Group(s): Smit, Tavernelli / Project(s): DD4 - S. Lyu, A. Pasquarello, Band alignment at β-Ga2O3/III-N (III = Al, Ga) interfaces through hybrid functional calculations, Applied Physics Letters 117, 102103 (2020). [Open Access URL]
Dataset on Materials Cloud.
Group(s): Pasquarello / Project(s): DD4 - S. M. Moosavi, A. Nandy, K. M. Jablonka, D. Ongari, J. P. Janet, P. G. Boyd, Y. Lee, B. Smit, H. Kulik, Understanding the Diversity of the Metal-Organic Framework Ecosystem, Nature Communications 11, 4068 (2020). [Open Access URL]
Dataset on Materials Cloud.
Group(s): Smit / Project(s): DD4 - S. Falletta, J. Wiktor, A. Pasquarello, Finite-size corrections of defect energy levels involving ionic polarization, Physical Review B 102, 041115(R) (2020). [Open Access URL]
Dataset on Materials Cloud.
Group(s): Pasquarello / Project(s): DD4 - Z. Guo, F. Ambrosio, A. Pasquarello, Evaluation of photocatalysts for water splitting through combined analysis of surface coverage and energy-level alignment, ACS Catalysis 10, 13186–13195 (2020). [Open Access URL]
Dataset on Materials Cloud.
Group(s): Pasquarello / Project(s): DD4 - L. Talirz, S. Kumbhar, E. Passaro, A. V. Yakutovich, V. Granata, F. Gargiulo, M. Borelli, M. Uhrin, S. P. Huber, S. Zoupanos, C. S. Adorf, C. W. Andersen, O. Schütt, C. A. Pignedoli, D. Passerone, J. VandeVondele, T. C. Schulthess, B. Smit, G. Pizzi, N. Marzari, Materials Cloud, a platform for open computational science, Scientific Data 7, 299 (2020). [Open Access URL]
1st dataset on Materials Cloud.
2nd dataset on Materials Cloud.
Group(s): Marzari, Passerone, Pizzi, Schulthess, Smit, VandeVondele / Project(s): DD4, OSP, HPC - S. M. Moosavi, H. L. Xu, L. J. Chen, A. I. Cooper, B. Smit, Geometric landscapes for material discovery within energy-structure-function maps, Chemical Science 11, 5423–5433 (2020). [Open Access URL]
Dataset on Materials Cloud.
Group(s): Smit / Project(s): DD4 - G. Bauer, D. Ongari, D. Tiana, P. Gäumann, T. Rohrbach, G. Pareras, M. Tarik, B. Smit, M. Ranocchiari, Metal-organic frameworks as kinetic modulators for branched selectivity in hydroformylation, Nature Communications 11, 1059 (2020). [Open Access URL]
Dataset on Materials Cloud.
Group(s): Ranocchiari, Smit / Project(s): DD4 - M. Fumanal, G. Capano, S. Barthel, B. Smit, I. Tavernelli, Energy-based descriptors for photo-catalytically active metal–organic framework discovery, Journal of Materials Chemistry A 8, 4473 (2020). [Open Access URL]
Dataset on Materials Cloud.
Group(s): Smit, Tavernelli / Project(s): DD4 - K. S. Deeg, D. D. Borges, D. Ongari, N. Rampal, L. Talirz, A. V. Yakutovich, J. M. Huck, B. Smit, In Silico Discovery of Covalent Organic Frameworks for Carbon Capture, ACS Applied Materials & Interfaces 12, 21559–21568 (2020). [Open Access URL]
Dataset on Materials Cloud.
Group(s): Smit / Project(s): DD4 - F. Stein, J. Hutter, V. V. Rybkin, Double-Hybrid DFT Functionals for the Condensed Phase: Gaussian and Plane Waves Implementation and Evaluation, Molecules 25, 5174 (2020). [Open Access URL]
Dataset on Materials Cloud.
Group(s): Hutter / Project(s): DD4 - S. Falletta, P. Gono, Z. Guo, S. Kampouri, K. C. Stylianou, A. Pasquarello, Unraveling the synergy between metal-organic frameworks and co-catalysts in photocatalytic water splitting, Journal of Materials Chemistry A 8, 20493–20502 (2020). [Open Access URL]
Dataset on Materials Cloud.
Group(s): Pasquarello, Smit, Stylianou / Project(s): DD4 - M. Fumanal, C. Corminboeuf, B. Smit, I. Tavernelli, Optical absorption properties of metal-organic frameworks: solid state versus molecular perspective, Physical Chemistry Chemical Physics 22, 19512 (2020). [Open Access URL]
Dataset on Materials Cloud.
Group(s): Corminboeuf, Smit, Tavernelli / Project(s): DD4 - T. T. Duignan, G. K. Schenter, J. L. Fulton, T. Huthwelker, M. Balasubramanian, M. Galib, M. D. Baer, J. Wilhelm, J. Hutter, M. Del Ben, X. S. Zhao, C. J. Mundy, Quantifying the hydration structure of sodium and potassium ions: taking additional steps on Jacob's Ladder, Physical Chemistry Chemical Physics 22, 10641–10652 (2020). [Open Access URL]
Group(s): Hutter / Project(s): DD4 - G. Tocci, M. Bilichenko, L. Joly, M. Iannuzzi, Ab initio nanofluidics: disentangling the role of the energy landscape and of density correlations on liquid/solid friction, Nanoscale 12, 10994 (2020). [Open Access URL]
Group(s): Hutter / Project(s): HP3, DD4 - T. D. Kühne, M. Iannuzzi, M. Del Ben, V. V. Rybkin, P. Seewald, F. Stein, T. Laino, R. Z. Khaliullin, O. Schütt, F. Schiffmann, D. Golze, J. Wilhelm, S. Chulkov, M. H. Bani-Hashemian, V. Weber, M. Brštnik Urban ad Taillefumier, A. S. Jakobovits, A. Lazzaro, H. Pabst, T. Müller, R. Schade, M. Guidon, S. Andermatt, N. Holmberg, G. K. Schenter, A. Hehn, A. Bussy, F. Belleflamme, G. Tabacchi, A. Glöß, M. Lass, I. Bethune, C. J. Mundy, C. Plessl, M. Watkins, J. VandeVondele, M. Krack, J. Hutter, CP2K: An electronic structure and molecular dynamics software package - Quickstep: Efficient and accurate electronic structure calculations, The Journal of Chemical Physics 152, 194103 (2020). [Open Access URL]
Dataset on Materials Cloud.
Group(s): Hutter, Laino, VandeVondele / Project(s): DD4 - T. Porȩba, P. Macchi, N. Casati, Structural Variety of Alkali Hydrogen Maleates at High Pressure, Crystal Growth & Design 20, 4375–4386 (2020).
Group(s): Macchi / Project(s): DD4 - M. Ernst, A. Genoni, P. Macchi, Analysis of crystal field effects and interactions using X-ray restrained ELMOs, Journal of Molecular Structure 1209, 127975 (2020). [Open Access URL]
Group(s): Macchi / Project(s): DD4 - A. Genoni, P. Macchi, Quantum Crystallography in the Last Decade: Developments and Outlooks, Crystals 10, 473 (2020). [Open Access URL]
Group(s): Macchi / Project(s): DD4 - S. Racioppi, M. Andrzejewski, V. Colombo, A. Sironi, P. Macchi, Different Metallophilic Attitudes Revealed by Compression, Inorganic Chemistry 59, 2223 (2020). [Open Access URL]
Group(s): Macchi / Project(s): DD4 - G. Capano, F. A. Francesco, S. Kampouri, K. C. Stylianou, A. Pasquarello, B. Smit, On the electronic and optical properties of metal-organic frameworks: case study of MIL-125 and MIL-125-NH2, The Journal of Physical Chemistry C 7, 4065 (2020). [Open Access URL]
Group(s): Pasquarello, Smit, Stylianou / Project(s): DD4 - K. M. Jablonka, D. Ongari, S. M. Moosavi, B. Smit, Big-Data Science in Porous Materials: Materials Genomics and Machine Learning, Chemical Reviews 120, 8066–8129 (2020). [Open Access URL]
Group(s): Smit / Project(s): DD4 - S. M. Moosavi, K. M. Jablonka, B. Smit, The Role of Machine Learning in the Understanding and Design of Materials, Journal of the American Chemical Society 142, 20273 (2020). [Open Access URL]
Group(s): Smit / Project(s): DD4 - B. Valizadeh, T. N. Nguyen, S. Kampouri, D. T. Sun, M. D. Mensi, K. Stylianou, B. Smit, W. L. Queen, A novel integrated Cr(vi) adsorption-photoreduction system using MOF@polymer composite beads, Journal of Materials Chemistry A 8, 9629–9637 (2020). [Open Access URL]
Group(s): Smit / Project(s): DD4 - J. Maul, D. Ongari, S. M. Moosavi, B. Smit, A. Erba, Thermoelasticity of Flexible Organic Crystals from Quasi-harmonic Lattice Dynamics: The Case of Copper(II) Acetylacetonate, The Journal of Physical Chemistry Letters 11, 8543–8548 (2020). [Open Access URL]
Group(s): Smit / Project(s): DD4 - S. L. Anderson, D. Tiana, C. P. Ireland, G. Capano, M. Fumanal, A. Gladysiak, S. Kampouri, A. Rahmanudin, N. Guijarro, K. Sivula, K. C. Stylianou, B. Smit, Taking lanthanides out of isolation: tuning the optical properties of metal-organic frameworks, Chemical Science 11, 4164–4170 (2020). [Open Access URL]
Group(s): Smit / Project(s): DD4 - A. Ortega-Guerrero, M. Fumanal, G. Capano, B. Smit, From Isolated Porphyrin Ligands to Periodic Al-PMOF: A Comparative Study of the Optical Properties Using DFT/TDDFT, The Journal of Physical Chemistry C 124, 21751–21760 (2020). [Open Access URL]
Group(s): Smit / Project(s): DD4 - A. Ortega-Guerrero, M. Fumanal, G. Capano, I. Tavernelli, B. Smit, Insights into the Electronic Properties and Charge Transfer Mechanism of a Porphyrin Ruthenium-Based Metal-Organic Framework, Chemistry of Materials 32, 4194 (2020). [Open Access URL]
Group(s): Smit, Tavernelli / Project(s): DD4 - S. Sudan, A. Gładysiak, B. Valizadeh, J. Lee, K. C. Stylianou, Sustainable Capture of Aromatic Volatile Organic Compounds by a Pyrene-Based Metal-Organic Framework under Humid Conditions, Inorganic Chemistry 59, 9029 (2020).
Group(s): Stylianou / Project(s): DD4 - V. V. Rybkin, Sampling Potential Energy Surfaces in the Condensed Phase with Many-Body Electronic Structure Methods, Chemistry–A European Journal 26, 362 (2020).
Group(s): Hutter / Project(s): DD4 - D. Ongari, Y. M. Liu, B. Smit, Can Metal-Organic Frameworks Be Used for Cannabis Breathalyzers?, ACS Applied Materials & Interfaces 11, 34777–34786 (2019). [Open Access URL]
Dataset on Materials Cloud.
Group(s): Smit / Project(s): DD4 - A. Bouzid, P. Gono, A. Pasquarello, Reaction pathway of oxygen evolution on Pt(111) revealed through constant Fermi level molecular dynamics, Journal of Catalysis 375, 135–139 (2019). [Open Access URL]
Dataset on Materials Cloud.
Group(s): Pasquarello / Project(s): DD3, DD4 - A. Gładysiak, T. N. Nguyen, R. Bounds, A. Zacharia, G. Itskos, J. A. Reimer, K. C. Stylianou, Temperature-dependent interchromophoric interaction in a fluorescent pyrene-based metal-organic framework, Chemical Science 10, 6140–6148 (2019). [Open Access URL]
Group(s): Stylianou / Project(s): DD4 - S. Kampouri, K. C. Stylianou, Dual-Functional Photocatalysis for Simultaneous Hydrogen Production and Oxidation of Organic Substances, ACS Catalysis 9, 4247–4270 (2019).
Group(s): Stylianou / Project(s): DD4 - B. Meyer, S. Barthel, A. Mace, L. Vannay, B. Guillot, B. Smit, C. Corminboeuf, DORI Reveals the Influence of Noncovalent Interactions on Covalent Bonding Patterns in Molecular Crystals Under Pressure, The Journal of Physical Chemistry Letters 10, 1482–1488 (2019). [Open Access URL]
Dataset on Materials Cloud.
Group(s): Corminboeuf, Smit / Project(s): DD1, DD4 - T. Musso, Atomistic Simulations in Surface Chemistry to Interpret Scanning Probe Microscopy Images: A Short Review, CHIMIA 73, 294–298 (2019). [Open Access URL]
Group(s): Hutter / Project(s): DD4 - J. Wiktor, A. Pasquarello, Electron and Hole Polarons at the BiVO4-Water Interface, ACS Applied Materials & Interfaces 11, 18423–18426 (2019). [Open Access URL]
Dataset on Materials Cloud.
Group(s): Pasquarello / Project(s): DD4 - S. L. Anderson, P. G. Boyd, A. Gładysiak, T. N. Nguyen, R. G. Palgrave, D. Kubicki, L. Emsley, D. Bradshaw, M. J. Rosseinsky, B. Smit, K. C. Stylianou, Nucleobase pairing and photodimerization in a biologically derived metal-organic framework nanoreactor, Nature Communications 10, 1612 (2019). [Open Access URL]
Group(s): Smit, Stylianou / Project(s): DD4 - S. Shyshkanov, T. N. Nguyen, F. M. Ebrahim, K. C. Stylianou, P. J. Dyson, In Situ Formation of Frustrated Lewis Pairs in a Water-Tolerant Metal-Organic Framework for the Transformation of CO2, Angewandte Chemie International Edition 58, 5371–5375 (2019).
Group(s): Stylianou / Project(s): DD4 - Z. Guo, F. Ambrosio, A. Pasquarello, Extrinsic Defects in Amorphous Oxides: Hydrogen, Carbon, and Nitrogen Impurities in Alumina, Physical Review Applied 11, 024040 (2019). [Open Access URL]
Dataset on Materials Cloud.
Group(s): Pasquarello / Project(s): DD3, DD4 - A. Mace, S. Barthel, B. Smit, Automated Multiscale Approach To Predict Self-Diffusion from a Potential Energy Field, Journal of Chemical Theory and Computation 15, 2127–2141 (2019). [Open Access URL]
Dataset on Materials Cloud.
Group(s): Smit / Project(s): DD4 - P. Oberholzer, E. Tervoort, A. Bouzid, A. Pasquarello, D. Kundu, Oxide versus Nonoxide Cathode Materials for Aqueous Zn Batteries: An Insight into the Charge Storage Mechanism and Consequences Thereof, ACS Applied Materials & Interfaces 11, 674–682 (2019).
Group(s): Pasquarello / Project(s): VP2, DD3, DD4 - F. M. Ebrahim, T. N. Nguyen, S. Shyshkanov, A. Gładysiak, P. Favre, A. Zacharia, G. Itskos, P. J. Dyson, K. C. Stylianou, Selective, Fast-Response, and Regenerable Metal–Organic Framework for Sampling Excess Fluoride Levels in Drinking Water, Journal of the American Chemical Society 141, 3052 (2019).
Group(s): Stylianou / Project(s): DD4 - A. Gładysiak, T. N. Nguyen, M. Spodaryk, J. Lee, J. B. Neaton, A. Züttel, K. C. Stylianou, Incarceration of Iodine in a Pyrene-Based Metal–Organic Framework, Chemistry–A European Journal 25, 501–506 (2019).
Group(s): Stylianou / Project(s): DD4 - T. Poreba, M. Ernst, D. Zimmer, P. Macchi, N. Casati, Pressure-Induced Polymerization and Electrical Conductivity of a Polyiodide, Angewandte Chemie International Edition 58, 6625–6629 (2019).
Group(s): Macchi / Project(s): DD4 - R. Scatena, Y. T. Guntern, P. Macchi, Electron Density and Dielectric Properties of Highly Porous MOFs: Binding and Mobility of Guest Molecules in Cu3(BTC)2 and Zn3(BTC)2, Journal of the American Chemical Society 141, 9382–9390 (2019). [Open Access URL]
Group(s): Macchi / Project(s): DD4 - M. Pizzochero, F. Ambrosio, A. Pasquarello, Picture of the wet electron: a localized transient state in liquid water, Chemical Science 10, 7442–7448 (2019). [Open Access URL]
Dataset on Materials Cloud.
Group(s): Pasquarello, Yazyev / Project(s): DD4 - P. G. Boyd, A. Chidambaram, E. García-Díez, C. P. Ireland, T. D. Daff, R. Bounds, A. Gładysiak, P. Schouwink, S. M. Moosavi, M. M. Maroto-Valer, J. A. Reimer, J. A. R. Navarro, T. K. Woo, S. Garcia, K. C. Stylianou, B. Smit, Data-driven design of metal-organic frameworks for wet flue gas CO2 capture, Nature 576, 253–256 (2019). [Open Access URL]
Dataset on Materials Cloud.
Group(s): Smit, Stylianou / Project(s): DD4 - A. Gładysiak, S. M. Moosavi, L. Sarkisov, B. Smit, K. C. Stylianou, Guest-dependent negative thermal expansion in a lanthanide-based metal-organic framework, CrystEngComm 21, 5292–5298 (2019). [Open Access URL]
Group(s): Smit, Stylianou / Project(s): DD4 - D. Ongari, A. V. Yakutovich, L. Talirz, B. Smit, Building a Consistent and Reproducible Database for Adsorption Evaluation in Covalent-Organic Frameworks, ACS Central Science 5, 1663–1675 (2019). [Open Access URL]
Dataset on Materials Cloud.
Group(s): Smit / Project(s): DD4 - M. A. Syzgantseva, C. P. Ireland, F. M. Ebrahim, B. Smit, O. A. Syzgantseva, Metal Substitution as the Method of Modifying Electronic Structure of Metal-Organic Frameworks, Journal of the American Chemical Society 141, 6271–6278 (2019). [Open Access URL]
Group(s): Smit / Project(s): DD4 - S. M. Moosavi, A. Chidambaram, L. Talirz, M. Haranczyk, K. C. Stylianou, B. Smit, Capturing chemical intuition in synthesis of metal-organic frameworks, Nature Communications 10, 539 (2019). [Open Access URL]
Dataset on Materials Cloud.
Group(s): Smit, Stylianou / Project(s): DD4 - D. Ongari, P. G. Boyd, O. Kadioglu, A. K. Mace, S. Keskin, B. Smit, Evaluating charge equilibration methods to generate electrostatic fields in nanoporous materials, Journal of Chemical Theory and Computation 15, 382 (2019). [Open Access URL]
Dataset on Materials Cloud.
Group(s): Smit / Project(s): DD4 - Z. Guo, F. Ambrosio, P. Gono, A. Pasquarello, Alignment of redox levels at semiconductor-water interfaces, Chemistry of Materials 30, 94 (2018).
Group(s): Pasquarello / Project(s): VP2, DD3, DD4 - F. Ambrosio, J. Wiktor, A. Pasquarello, pH-dependent catalytic reaction pathway for water splitting at the BiVO4-water interface from the band alignment, ACS Energy Letters 3, 829 (2018).
Group(s): Pasquarello / Project(s): VP2, DD4 - F. Ambrosio, J. Wiktor, A. Pasquarello, pH-dependent surface chemistry from first principles: Application to the BiVO4(010)-water interface, ACS Applied Materials & Interfaces 10, 10011 (2018).
Group(s): Pasquarello / Project(s): VP2, DD4 - A. Bouzid, A. Pasquarello, Atomic-scale simulation of electrochemical processes at electrode/water interfaces under referenced bias potential, The Journal of Physical Chemistry Letters 9, 1880 (2018).
Group(s): Pasquarello / Project(s): VP2, DD3, DD4 - P. Gono, J. Wiktor, F. Ambrosio, A. Pasquarello, Surface polarons reducing overpotentials of the oxygen evolution reaction, ACS Catalysis 8, 5847 (2018).
Group(s): Pasquarello / Project(s): VP2, DD3, DD4 - F. Ambrosio, Z. Guo, A. Pasquarello, Absolute energy levels of liquid water, The Journal of Physical Chemistry Letters 9, 3212 (2018).
Group(s): Pasquarello / Project(s): VP2, DD4 - Z. Guo, F. Ambrosio, A. Pasquarello, Hole diffusion across leaky amorphous TiO2 coating layers for catalytic water splitting at photoanodes, Journal of Materials Chemistry A 6, 11804 (2018).
Group(s): Pasquarello / Project(s): VP2, DD4 - J. Wiktor, F. Ambrosio, A. Pasquarello, Role of polarons in water splitting: The case of BiVO4, ACS Energy Letters 3, 1693 (2018).
Group(s): Pasquarello / Project(s): VP2, DD4 - J. Wiktor, F. Ambrosio, A. Pasquarello, Mechanism suppressing charge recombination at iodine defects in CH3NH3PbI3 by polaron formation, Journal of Materials Chemistry A 6, 16863 (2018).
Group(s): Pasquarello / Project(s): VP2, DD4 - J. Wiktor, I. Reshetnyak, M. Strach, M. Scarongella, R. Buonsanti, A. Pasquarello, Sizable excitonic effects undermining the photocatalytic efficiency of β-Cu2V2O7, The Journal of Physical Chemistry Letters 9, 5698 (2018).
Group(s): Buonsanti, Pasquarello / Project(s): VP2, PP7, DD4 - F. Ambrosio, A. Pasquarello, Reactivity and energy level of a localized hole in liquid water, Physical Chemistry Chemical Physics 20, 30281 (2018).
Group(s): Pasquarello / Project(s): VP2, DD4 - L. Talirz, C. A. Pignedoli, Electronic Structure of Atomically Precise Graphene Nanoribbons, in Handbook of Materials Modeling: Applications: Current and Emerging Materials, W. Andreoni and S. Yip, eds. (Springer, Cham, 2018), pp. 1–35.
Group(s): Passerone, Smit / Project(s): DD3, DD4 - S. Barthel, E. V. Alexandrov, D. M. Proserpio, B. Smit, Distinguishing Metal–Organic Frameworks, Crystal Growth & Design 18, 1738–1747 (2018). [Open Access URL]
Group(s): Smit / Project(s): DD4 - E. Braun, Y. Lee, S. M. Moosavi, S. Barthel, R. Mercado, I. A. Baburin, D. M. Proserpio, B. Smit, Generating carbon schwarzites via zeolite-templating, Proceedings of the National Academy of Science of the USA 115, E8116–E8124 (2018). [Open Access URL]
Dataset on Materials Cloud.
Group(s): Smit / Project(s): DD4 - S. Kampouri, T. N. Nguyen, C. P. Ireland, B. Valizadeh, F. M. Ebrahim, G. Capano, D. Ongari, A. Mace, N. Guijarro, K. Sivula, A. Sienkiewicz, L. Forró, B. Smit, K. C. Stylianou, Photocatalytic hydrogen generation from a visible-light responsive metal-organic framework system: the impact of nickel phosphide nanoparticles, Journal of Materials Chemistry A 6, 2476–2481 (2018).
Group(s): Smit, Stylianou / Project(s): DD4 - Y. Lee, S. D. Barthel, P. Dłotko, S. M. Moosavi, K. Hess, B. Smit, High-Throughput Screening Approach for Nanoporous Materials Genome Using Topological Data Analysis: Application to Zeolites, Journal of Chemical Theory and Computation 14, 4427–4437 (2018). [Open Access URL]
Group(s): Smit / Project(s): DD4 - R. Mercado, R. Fu, A. V. Yakutovich, L. Talirz, M. Haranczyk, B. Smit, In Silico Design of 2D and 3D Covalent Organic Frameworks for Methane Storage Applications, Chemistry of Materials 30, 5069–5086 (2018). [Open Access URL]
Dataset on Materials Cloud.
Group(s): Smit, Pizzi / Project(s): DD4, OSP - S. M. Moosavi, P. G. Boyd, L. Sarkisov, B. Smit, Improving the Mechanical Stability of Metal–Organic Frameworks Using Chemical Caryatids, ACS Central Science 4, 832–839 (2018). [Open Access URL]
Dataset on Materials Cloud.
Group(s): Smit / Project(s): DD4 - M. Witman, S. Ling, P. Boyd, S. Barthel, M. Haranczyk, B. Slater, B. Smit, Cutting Materials in Half: A Graph Theory Approach for Generating Crystal Surfaces and Its Prediction of 2D Zeolites, ACS Central Science 4, 235–245 (2018). [Open Access URL]
Group(s): Smit / Project(s): DD4 - T. N. Nguyen, F. M. Ebrahim, K. C. Stylianou, Photoluminescent, upconversion luminescent and nonlinear optical metal-organic frameworks: From fundamental photophysics to potential applications, Coordination Chemistry Reviews 377, 259–306 (2018). [Open Access URL]
Group(s): Stylianou / Project(s): DD4 - T. N. Nguyen, S. Kampouri, B. Valizadeh, W. Luo, D. Ongari, O. M. Planes, A. Züttel, B. Smit, K. C. Stylianou, Photocatalytic Hydrogen Generation from a Visible-Light-Responsive Metal–Organic Framework System: Stability versus Activity of Molybdenum Sulfide Cocatalysts, ACS Applied Materials & Interfaces 10, 30035–30039 (2018). [Open Access URL]
Group(s): Smit, Stylianou / Project(s): DD4 - S. Kampouri, T. N. Nguyen, M. Spodaryk, R. G. Palgrave, A. Züttel, B. Smit, K. C. Stylianou, Concurrent Photocatalytic Hydrogen Generation and Dye Degradation Using MIL-125-NH2 under Visible Light Irradiation, Advanced Functional Materials 28, 1806368 (2018).
Group(s): Smit, Stylianou / Project(s): DD4