Using machine learning to study the microscopic behavior of a solid-state electrolyte
By Nicola Nosengo - NCCR MARVEL
Solid-state batteries could be a game changer for electric vehicles and for other applications. Not only can they pack more energy in less space than current lithium-ion liquid-electrolyte batteries, but they are safer, charge faster, and last longer. Scientists and engineers are still looking for the best materials to use as solid electrolytes, and one that is getting a lot of attention is lithium thiophosphate, or Li3PS4, where lithium ions are arranged in a solid matrix of tetrahedra, each made of one phosphorus and four sulfur atoms.
“There is a rich literature on this material,” says Federico Grasselli, a scientist in Michele Ceriotti’s lab at EPFL and co-author of a new computational study that was just published in Chemistry of Materials. “The first experimental works date back to the 1980s, and there have been quite a few ab initio computational studies, but the emphasis on the study of its properties has only increased recently because of the interest in solid-state batteries, and some aspects are still not understood”.
Scientists are particularly interested in the material’s α phase, that appears above 746 K (472 °C) and is characterized by a staggered order of the tetrahedra formed by the phosphorus and sulfur atoms. This phase, the one where the material shows the highest conductivity, has only been discovered experimentally in 2019 and there are few computational studies on it. And yet its full description is key to explain the mechanism of charge transport.
“There are no empirical potentials (i.e. numerical descriptions of the interactions between atoms upon which simulations can be based) that accurately described the material, particularly the α phase,” says Davide Tisi, also in the COSMO lab and another co-author, together with Lorenzo Gigli and Michele Ceriotti. “Doing ab initio simulations (i.e., based on a quantum-mechanical description of the electrons) is exceedingly costly and does not allow to simulate large cells”. So, the scientists resorted to creating potentials through machine learning, which offers a way to study the material in a systematic way while striking a balance between accuracy and economy of calculations. “Machine learning allowed us to find potentials that are not as fast as empirical ones but can easily simulate hundreds or thousands of atoms for a sufficiently long time”, they explain.
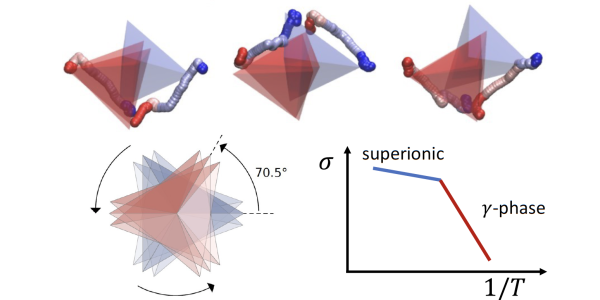
As a first step, the scientists had to create a training set that would allow an algorithm to learn its way to accurate potentials. They combined simulations based on the
Car-Parrinello method and DFT calculation with the widely used PBEsol functional to create an initial set of structures and calculate their properties. “This gave us some initial snapshots of the material in different phases and at different temperatures, and we used them to create a first potential with machine learning”, says Tisi. “Then we ran new simulations and obtained further snapshots covering more temperatures”. They repeated these steps several times, always combining molecular dynamics, DFT and machine learning and extending the data set until it included thousands of structures, and the model was sufficiently accurate.
“Towards the end we were able to run simulations beyond the melting temperature of the material (approximatively 1,000 K - or 726 °C - at ambient pressure), to have reliable results also in these extreme conditions” says Grasselli. The whole dataset is now available for other researchers on the Materials Cloud.
In the end, the potentials developed through machine learning allowed the team to simulate the mechanisms of charge transport in lithium thiophosphate in unprecedented detail.
The scientists now have quite a precise picture of how the material goes from a state where it conducts few lithium ions (γ, the most stable one at low temperature) to another polymorph (the β phase) that conducts better, until it reaches the α phase that is the most conductive one. What has not really been clear until now is how the diffusion of lithium ions happens on a microscopic timescale. “One big question was the role played by the rotation of the PS4 tetrahedra” says Tisi. Scientists know that these tetrahedra can undergo rotations, or flips, that play a part in the phase transition. But they were wondering if they also carry lithium ions with them as they flip, activating a sort of conveyor belt for ions - the so-called called “paddle-wheel effect”. “What we found is that the timescale of the flips is actually much slower than the transport of ions, and the flips are not frequent enough to contribute to charge transport,” Tisi explains. “The flips do cause a structural phase change, and the new structure favors the transport of ions, but they do not contribute directly to it”
Another important finding is that the correlation effects between lithium atoms are important for understanding charge transport. While common approximations treat every atom as independent from the others, an accurate description of ionic conduction requires a more complete model that accounts for the fact that when a lithium ion moves, another one tends to follow its trail.
The group is now perfecting the study of this material by adding an analysis of thermal transport, which will be the topic of a new publication (for a preprint, see here). “A common problem of these materials is that, precisely because they are good charge conductors, during their recharging phase they can heat because of the Joule effect,” says Grasselli. “But excessive heating can cause them to crack and deform, compromising their efficiency in batteries”.
Reference
Gigli L., Tisi D., Grasselli F. and Ceriotti M..,"Mechanism of Charge Transport in Lithium Thiophosphate", Chemistry of Materials 36, 3, 1482–1496 (2024) DOI: https://doi.org/10.1021/acs.chemmater.3c02726
Low-volume newsletters, targeted to the scientific and industrial communities.
Subscribe to our newsletter