Graph neural network parametrized potentials describe intermolecular interactions
By Carey Sargent, EPFL, NCCR MARVEL
Computer-based simulations allow for detailed investigation of chemical systems, but performing them requires accurate descriptions of intermolecular forces. Techniques such as ab initio methods or density functional theory can provide this essential information, but the computational cost of such approaches limits their application to large systems. This is particularly true for biologically relevant systems.
Because of this, empirical methods such as force fields (FF) have become an established tool for simulating large and complex molecular systems. However, parametrization of FF is time consuming, and has generally been mostly based on experimental data, which is often scarce. In recent years, efforts have focused on automatizing FF parametrization and moving towards fitting FF against readily accessible quantum-mechanical reference data such as torsion profiles or interaction components, as well as efforts to extract FF parameters directly from electron densities. Other work has investigated graph neural networks (GNN) and graph-convolutional neural networks (GCNN) in combination with automatic differentiation as a way of parametrizing FF.
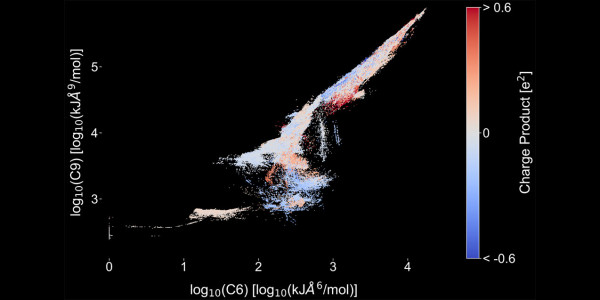
Figure 1: Predicted C6 and C9 parameters by the IPAd+c model on log scale for all atom pairs in the data set of intermolecular potentials of crystals (11,489 molecules). The color indicates the strength and sign of the electrostatic interaction (blue = attractive, red = repulsive).
In the paper “Regularized by Physics: Graph Neural Network Parametrized Potentials for the Description of Intermolecular Interactions,” recently published in the Journal of Chemical Theory and Computation, a team at ETHZ led by Prof. Sereina Riniker, Associate Professor of Computational Chemistry at the Department of Chemistry and Applied Biosciences, built on those developments to propose an alternative strategy for parametrizing intermolecular interactions.
The approach uses machine learning and gradient-descent based optimization, but keeps a functional form founded in physics: the strategy, the researchers say, can be seen as a generalization of commonly used FF definitions and parametrization procedures, but also describes the regularization of ML models through physics. Using graph neural networks together with automatic differentiation to parametrize physically motivated models to potential-energy surfaces allows for full automatization as well as broad applicability in the chemical space.
The scientists demonstrated the strength of their method by using it to parametrize an isotropic-pairwise-additive FF (IPA model) as well as a polarizable FF (ANA model). They first assessed model performance on intermolecular interaction energies of dimers in the gas phase. They found that both approaches perform as well as or better than comparable methods on several common benchmark data sets, while requiring little computational effort or human intervention. Importantly, parametrization was carried out exclusively with respect to the PES of reference QM calculations without the addition of experimental data.
A further optimized model, IPAd+c , was then applied to the calculation of condensed-phase properties such as lattice energies and pure liquid properties. They found that it—a fixed-charge FF parametrized on intermolecular potentials from DFT calculations—can provide consistent results over a wide range of systems, from dimer interaction potentials in a vacuum to pure liquid properties. The researchers said that while a completely classical description of molecular forces has obvious limitations such as an inability to model bond formation or breaking, it might still be preferable to semi-empirical methods: implicitly describing, e.g., the electron distribution through atomic multipoles offers a promising alternative to semi-empirical models because it provides a comparable level of accuracy at only a fraction of the computational cost.
“The presented approach accelerates and simplifies the parametrization process of classical FF and can take advantage of large data sets,” the researchers said in the paper. “In combination with ML based techniques, such as the presented GNN-based atom-typing, the best of both worlds can be united: a universal optimization toolkit combined with robust and physically-constrained models.”
References:
M. Thürlemann, L. Böselt, S. Riniker. Regularized by Physics: Graph Neural Network Parametrized Potentials for the Description of Intermolecular Interactions. J. Chem. Theory Comput. 2023, 19, 2, 562–579.
Low-volume newsletters, targeted to the scientific and industrial communities.
Subscribe to our newsletter