New organocatalysts database to drive reaction optimization methods in organic synthesis
Along with transition-metal catalysis and biocatalysis, organocatalysis – the use of small (chiral) organic molecules as catalysts for (stereoselective) reactions – is a pillar of synthetic chemistry, with a huge variety of conceptually and chemically different catalyst classes.
Computational techniques have offered invaluable insight into reaction mechanisms and helped perform a fine-tuning of catalyst structure, but have failed at becoming truly predictive: they are limited to case-by-case studies and dominated by trial-and-error practices.
This is slowly changing, as more sophisticated artificial intelligence-based strategies are being implemented in organic synthesis. Such data-driven tools rely, however, on having access to diverse and systematic datasets, which are currently missing for organocatalysis. As a result, reaction optimization is often limited to testing closely related analogues of known “privileged” catalysts. The design of new species from scratch is rarely attempted.
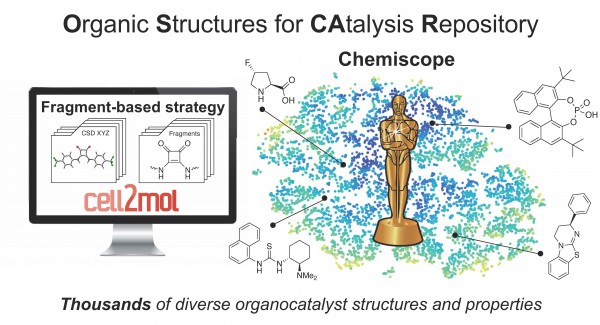
The lack of databases facilitating the exploration of wider regions of catalyst space and the optimization of reaction properties is due to the difficulty of viewing organocatalysts in a modular fashion and fragmenting them into building blocks. There are currently no general fragment-oriented strategies or platforms for catalyst comparison and the identification of chemically diverse functional units that could serve as the starting point for new catalyst designs.
In the paper “OSCAR: an extensive repository of chemically and functionally diverse organocatalysts,” Simone Gallarati and the team led by Professor Clemence Corminboeuf, head of the Laboratory for Computational Molecular Design at EPFL, address this problem with the construction of OSCAR (Organic Structures for CAtalysis Repository). This dataset automatically contains thousands of organic molecules that have been mined from the literature and from the Cambridge Crystallographic Database, and enriched with species generated in a combinatorial fashion by recombining molecular building blocks.
The result is a map that could help researchers navigate organocatalyst space and enable informed catalyst design. It’s also the starting point for a multitude of fragment-based reaction optimization methods.
“OSCAR covers a wide region of catalyst space with incomparable chemical diversity, and includes a selection of steric and electronic molecular descriptors useful for catalytic properties estimation and performance prediction,” the researchers said.
The paper outlines how the dataset is curated using cell2mol and describes the strategy behind the construction of two “supersets” for covalent and non-covalent catalysis, OSCAR!(NHC) and OSCAR!(DHBD). The former consists of more than 8,000 N-heterocyclic carbenes, while the latter contains more than 1.5 million dual-hydrogen-bond donors. The approach is transferable to other catalyst classes, suggesting that OSCAR could be extended further.
All structures and properties are publicly available on the Materials Cloud for interactive visualization with Chemiscope developed by Prof. Michele Ceriotti's Laboratory of Computational Science and Modelling (COSMO) at EPFL. The researchers aim to use the structure database to perform evolutionary experiments and find optimal catalysts for specific reactions.
“In general, we believe OSCAR will be valuable to the wider computational catalysis community to develop machine learning models for the prediction of organocatalyst performance and the optimization of reaction properties,” Gallarati said.
References:
S. Gallarati, C. Corminboeuf, P. van Gerwen, R. Laplaza, A. Fabrizio, S. Vela, OSCAR: An Extensive Repository of Chemically and Functionally Diverse Organocatalysts, Chem. Sci., 2022
DOI: https://doi.org/10.1039/D2SC04251G
Low-volume newsletters, targeted to the scientific and industrial communities.
Subscribe to our newsletter