Machine-learning models of matter beyond interatomic potentials
by Carey Sargent, NCCR MARVEL, EPFL
Combining electronic structure calculations and machine learning (ML) techniques has become a common approach in the atomistic modelling of matter. Using the two techniques together has allowed researchers, for instance, to create models that use atomic coordinates as the only inputs to inexpensively predict any property that can be computed by the first-principles calculations that had been used to train them.
While the earliest and by now most advanced efforts have focused on using predictions of total energies and atomic forces to construct interatomic potentials, more recent efforts have targeted additional properties of crystals and molecules such as ionization energies, NMR chemical shieldings, dielectric response properties and charge density. In the paper Learning the electronic density of states in condensed matter, Ceriotti and colleagues focus on the electronic density of states (DOS), another quantity that underlies many useful materials properties, some of which can be observed experimentally.
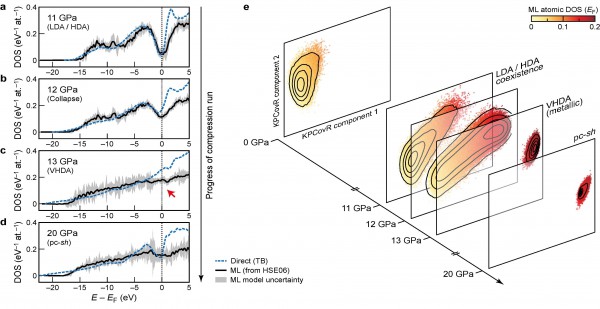
Fig. 1: Electronic fingerprints of structural transitions. From V.L. Deringer, N. Bernstein, G. Csányi, et al. Origins of structural and electronic transitions in disordered silicon. Nature 589, 59–64 (2021)
The DOS is essentially the number of different states that electrons can occupy at a particular energy level and can be used, for instance, to calculate the electronic contribution to heat capacity in metals and the density of free charge carriers in semiconductors. It is an indirect proxy for properties such as the energy band gap, the band energy and the optical absorption spectrum.
“Predicting the DOS is an interesting exercise in itself because it is essentially the simplest possible description of the electronic structure beyond the ground state picture,” Ceriotti said. “It’s also useful because there are many properties that you can compute starting from the DOS, making it a great example of how the next generation of ML models can be used in a similar way as electronic structure calculations, using them in an indirect way to compute intermediate quantities that can then be easily processed to evaluate properties that are harder to learn directly.”
In developing the model, the group looked to assure transferability across different phases as well as scalability to large system sizes. Their ultimate approach, which looks at how different atomic configurations affect the distribution of energy levels, meets these goals—it was able to learn and predict DFT-computed DOS for a diverse data set of silicon structures, covering a broad range of thermodynamic conditions and different phases. It also scales linearly, rather than with the cube of the number of atoms as with electronic structure calculations, making it applicable to large structures. Finally, the model allowed for an analysis of the local DOS, giving researchers the chance to examine the interplay between structural motifs and electronic structure.
The combination of transferability, and scalability of predictions to large system sizes, make the model applicable to address long-standing open questions in materials science. The new framework has already been used to elucidate the electronic properties of a 100’000-atoms simulation of amorphous silicon, undergoing a series of phase transitions when compressed to 20 Gpa, in a paper published in Nature today in collaboration with a team comprising researchers from Oxford, Cambridge, the US Naval Research Laboratory and Ohio University. The predicted DOS is also used to explain how the pressure-induced structural transformations couple to the electronic structure of the material.
Combining the new model with one of the well-established potential energy models also makes it possible to compute the electronic contributions to macroscopic properties such as the heat capacity of metals and to perform simulations that take into account finite-electronic-temperature effects – as demonstrated in another soon-to-be published article discussing the high-temperature properties of nickel. Indeed, the new model is a critical step towards MARVEL’s goal of developing integrated machine learning models that augment – and perhaps eventually replace – costly electronic structure calculations.
“There are other properties aside from the electron density of states, such as optical excitations, and NMR response, which we have been able to accurately predict with machine learning.” Ceriotti said. “If we can use them all in combination with cheap and accurate interatomic potentials it will allow us to describe all of the properties of materials with the same accuracy achieved with electronic structure calculation, but at a tiny fraction of the cost.”
References:
C. Ben Mahmoud, A. Anelli, G. Csányi, and M. Ceriotti, Learning the electronic density of states in condensed matter, Physical Review B 102, 235130 (2020). [Open Access URL]
V. L. Deringer, N. Bernstein, G. Csányi, C. Ben Mahmoud, M. Ceriotti, M. Wilson, D. A. Drabold, S. R. Elliott, Origins of structural and electronic transitions in disordered silicon, Nature 589, 59 (2021). [Open Access URL] / Dataset on Zenodo.
Low-volume newsletters, targeted to the scientific and industrial communities.
Subscribe to our newsletter