“Amon”-based ML approach excels in modelling quantum properties of a wide range of systems
by Carey Sargent, NCCR MARVEL, EPFL
The exploration of molecules through the calculation of numerical solutions to the fundamental quantum mechanical laws of nature helps researchers better understand the properties of existing or theoretical compounds—solving the Schrödinger equation allows researchers to compute the electronic structure of materials and molecules and from this, derive all ground-state properties. The associated computational cost of approximating these solutions however, as well as the huge number of theoretically possible stable compounds, means that comprehensive in-silico screening is still prohibitively expensive.
Researchers have looked to machine learning to lessen this computational cost by training models to infer data points from sets of training data rather than actually calculating each one. They suffer from severe limitations however in that the models can then only be applied to domains that resemble the training data. That is, conventional machine learning protocols rely on a pre-defined dataset that typically has a biased origin and is then sub-sampled at random or through active learning for training and testing.
The approach is linked to two significant drawbacks—scalability and transferability. In terms of scalability, limits are rapidly reached because the composition and size of the training compounds must nonetheless match the number of compositional elements and/or the size of the system being investigated. The combinatorial explosion of the possible number of compounds as a function of atom number and types means that prohibitively large training data sets are rapidly required.
In terms of transferability, the lack of generalization to chemistries that were not already incorporated into the training set and, on the flip side, the overfitting to the chemistries that were, significantly limits the broad, robust applicability of ML models. The authors note that the sampling of compounds found in nature introduces significant bias towards atomic environments or bonding patterns that have been favored by the particular free energy reaction pathways on planet earth. They were in turn favored because of boundary conditions such as the abundance of a given element, planetary and ambient conditions and evolutionary chemistries. Models, however, are only useful to the extent that they allow for reasonable levels of generalization.
While some ab initio methods have looked to address the issues of transferability and scalability, they typically trade accuracy or transferability for speed. And while transferable by design, popular “cheminformatics” models—models based on extended connectivity fingerprint descriptors—have not yet been successfully applied to quantum properties.
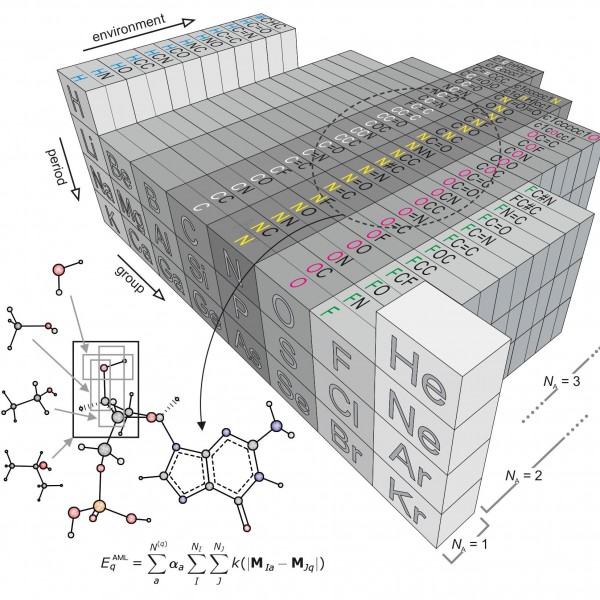
Figure 1: “Amons” —a compositional extension of the periodic table
The issue inspired researchers Anatole von Lilienfeld and Bing Huang at the University of Basel to take a new approach based on so-called amons, or Atom-in-Molecule-on. The method involves on-the-fly training using reference instances that are selected by local similarity measures from a dictionary of a series of small molecular building blocks which systematically grow in size.Since such building blocks repeat throughout the chemical space of larger query compounds, they can be viewed as indistinguishable entities, each representing an amon. The AML approach accounts for chemical environments by building on Mendeleev's periodic table, allowing for the systematic reconstruction of many chemistries from local building blocks.
The AML models make it possible to enumerate completely the constituting groups no matter how large and diverse the query through an approach that infers the energy of any query compound based on a linear combination of properly weighted reference results—coming from quantum chemistry calculations or experiments—for the building blocks that make it up.
The approach to adding the amons together is also critical. Large query molecules could be broken up by, for example, cascades of bond separating reactions, to obtain increasingly smaller and more common molecular fragments. Like this, however, the summed energy will increasingly deviate from that of the query. To systematically control such errors, the researchers used a reverse procedure: starting from very small amons, increasingly larger ones, representing fragments, are included and this results in increasingly more accurate ML models. Like this, they are able to minimize the size of the training set by selecting only the most relevant amons, that is those small fragments that retain the local chemical environments that are encoded in the coordinates of the query molecule.
The researchers tested the scalability and transferability of their approach by looking at total energy predictions for two dozen large, important biomolecules, including cholesterol, cocaine, and vitamin D2 from their model. The results indicated systematic improvement of predictive accuracy, with errors reaching those typically associated with bond-counting, DFT, or experimental thermochemistry for amons with 3, 5, or 7 heavy atoms, respectively. In smaller molecules with rigid and strain-less structure and homogeneous chemical environments of the constituting atoms—for example, vitamin B3 with only 9 heavy atoms—the prediction error decreased faster with amon size than for more complex molecules and reached reaching chemical accuracy with only about 20 amons in total.
The results suggest that a finite set of small to medium sized building block molecules is sufficient for the automatized generation of quantum machine learning models that feature favorable combinations of efficiency, accuracy, scalability, and transferability. The amon dictionary allows researchers to use such models to rapidly estimate ground state quantum properties of large and real materials with chemical accuracy. This means, the researchers said, that atomistic simulation protocols reaching the accuracy of high-level reference methods, such as post-Hartree-Fock or quantum Monte Carlo, for large systems are no longer prohibitive in the foreseeable future.
Reference:
Huang, B., von Lilienfeld, O.A. Quantum machine learning using atom-in-molecule-based fragments selected on the fly. Nature Chemistry 12, 945 (2020). [Open Access URL] / Dataset on Zenodo.
Low-volume newsletters, targeted to the scientific and industrial communities.
Subscribe to our newsletter