MARVEL Junior Seminar — September 2023
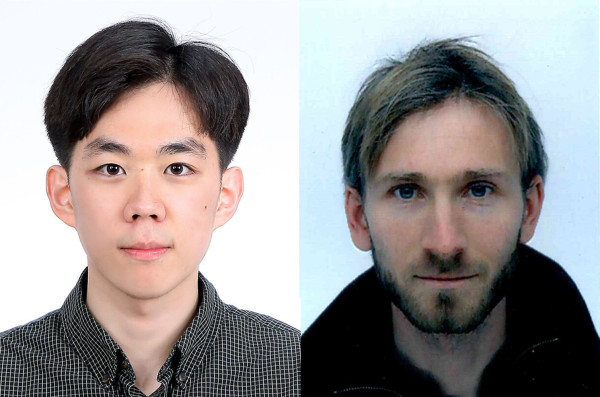
Each seminar consists of two presentations of 25 minutes each, allowing to present on a scientific question in depth, followed by time for discussion. The discussion is facilitated and timed by the chair.
Onsite participation
11:45 — Pizzas will be served in the MED building atrium, second floor
12:15 — Seminars take place in EPFL room Coviz2 (MED 2 1124)
Online participation
Starting at 12:15:
https://epfl.zoom.us/j/68368776745
Password: 1923
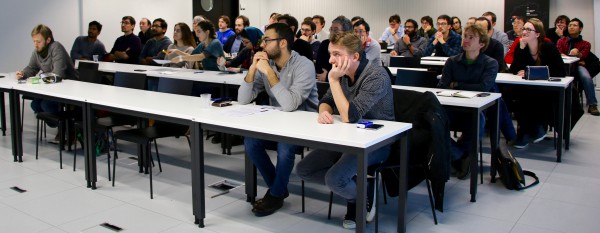
Abstracts
Talk 1 — Robustness of local and component-wise predictions in machine learning models
Sanggyu Chong
Laboratory of Computational Science and Modeling - COSMO, EPFL
Machine learning (ML) models for molecules and materials commonly rely on the decomposition of a global target quantity into local contributions from atoms, and/or distinct prediction components that describe a conceptually separable portion of the property of interest (e.g. body orders, short range + long range). This approach is convenient from a computational perspective, and also allows for the post-hoc interpretation of how different atoms/prediction components contribute to the complicated macroscopic properties. However, even though there exist such practical justifications, only the global quantity is rigorously defined, and it remains unclear to what extent the local or component-wise predictions of the model can be trusted. To this end, we introduce several flavors of “prediction rigidities”, which are quantitative metrics that allow one to assess how robust the local and component-wise predictions of ML models are. We investigate the dependence of these metrics on the aspects of model training, particularly the composition of training dataset, for a range of problems from simple toy models to real chemical systems. We present strategies to systematically enhance the prediction rigidity, which then leads to improvements in the robustness, interpretability, and transferability of ML models.
Talk 2 — Equivariant machine learning: A natural and highly data-efficient tool for predicting physical quantities
Martin Uhrin
Laboratory of Theory and Simulation of Materials - THEOS, EPFL
In this talk, I will give an introduction to group equivariant neural networks and outline how these differ from the more well-known multi-layer perceptrons. I will focus on showing how built-in symmetry awareness and other physically-motivated constraints make these models more data-efficient and transferable, allowing them to do more with fewer data
Two applications will be used as examples. The first involves predicting Hubbard U and V parameters using fully equivariant features of the on-site and intra-site electronic structure as inputs. I will show how this flexible model makes it trivial to express and perform learning on many-body representations. The model achieves excellent accuracy and transferability across a range of elements and enables a speedup of around 80x compared to using linear response. In the second example, I will show how we can predict tensorial properties, namely Born effective charges (rank 2) and Raman tensors (rank 3), using a single double-headed, equivariant graph neural network. Remarkably, this model can learn effectively from only one or two training structures and extrapolate to a dataset of 50. For both examples, I will show that the models can be easily defined in my JAX based python library `tensorial`, by formulating the input and output data in terms of irreducible representations of O(3).
Check the list of the next MARVEL Junior Seminars here.
Low-volume newsletters, targeted to the scientific and industrial communities.
Subscribe to our newsletter