MARVEL Junior Seminar — June 2018
The MARVEL Junior Seminars aim to intensify interactions between the MARVEL Junior scientists belonging to different research groups located at EPFL. The EPFL community interested in MARVEL research topics is very welcome to attend. We believe that these events will be central for establishing a vibrant community.
Each seminar consists of two presentations of 25 minutes each, allowing to present on a scientific question in depth, each presentation being followed by 10 minutes for discussion. The discussion is facilitated and timed by the chairperson of the day whose mission is to ensure active lively interactions between the audience and the speakers.
Pizza is served as of 11:45 in the MED hall (floor 0), and after the seminar at 13:30 you are cordially invited for coffee and dessert to continue discussion with the speakers.
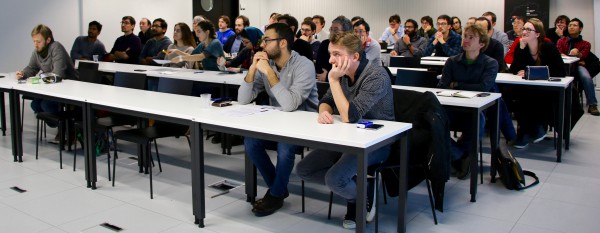
MARVEL Junior Seminar Organizing Committee — Francesco Ambrosio, Davide Campi, Edgar Engel, Gloria Capano, Michele Pizzochero, Quang Van Nguyen, Kun-Han Lin, Francesco Maresca and Nathalie Jongen
Check the list of the next MARVEL Junior Seminars here.
Abstract — Topological characterization of metals - Daniel Gosalbez Martinez
The topological classication of matter has been extended to include semimetallic phases that are characterized by the presence of topologically protected band degeneracies. The Weyl semimetals are the most popular, with isolated chiral degeneracies near the Fermi level and associated surface states called Fermi arcs. However, chiral degeneracies are ubiquitous and rarely isolated in the and structure of any spatial inversion (P) or time-reversal symmetry(T) broken system. In the case of metals, the Fermi surface sheets with nonzero Chern number inherit the topological nature of the chiral degeneracies. In this talk we present a review of all these new of topological phases, paying special attention to the classification of chiral degeneracies in type-I and type-II in composite Weyl nodes, and we will explore the consequences of having chiral degeneracies in metallic systems studying the surface states and surface resonances on bcc iron.
Abstract — Machine Learning for molecular materials: stability, properties and experimental observables - Felix Musil
Felix Musil1, Sandip De1, Federico M. Paruzzo2, Albert Hofstetter2, Yang Jack3, Joshua E. Campbell3, Graeme M. Day3, Lyndon Emsley2, Michele Ceriotti1.
1. Institut des Sciences et Ingénierie Chimiques, Ecole Polytechnique Fédérale de Lausanne (EPFL), 1015 Lausanne, Switzerland.
2. Institut des Sciences et Génie Matériaux, Ecole Polytechnique Fédérale de Lausanne (EPFL), 1015 Lausanne, Switzerland.
3. School of Chemistry, University of Southampton, Highfield, Southampton, UK.
Molecular crystals play an important role in several fields of science and technology. They frequently crystallize in different polymorphs with substantially different physical properties. To understand their structures, machine-learning (ML) methods have recently emerged as a way to overcome the need for expensive explicit high accuracy first-principle calculations. We show how a recently-developed ML framework [1] can be used to achieve sub-kJmol accuracy in the prediction of DFT lattice energies of pentacene and two azapentacene isomers [2], using only a few hundred reference configurations and provide a data-driven classification that is less biased and more flexible than typical heuristic rules.
At the same time, the elucidation of the structure of synthesized molecular crystals often requires theoretical inputs to interpret NMR spectra. We use a ML method based on local environments to accurately predict chemical shifts of different molecular solids and of different polymorphs within DFT accuracy [3], RMSE of 0.49 ppm (1H), 4.5ppm (13C), 13.3 ppm (14N), and 17.7 ppm (17O), and we demonstrate that the trained model is able to correctly determine structures of cocaine and the drug 4-[4-(2-adamantylcar- bamoyl)-5-tert-butylpyrazol-1-yl]benzoic acid in a chemical shift based NMR crystallography approach.
Low-volume newsletters, targeted to the scientific and industrial communities.
Subscribe to our newsletter