MARVEL Junior Seminar — December 2023
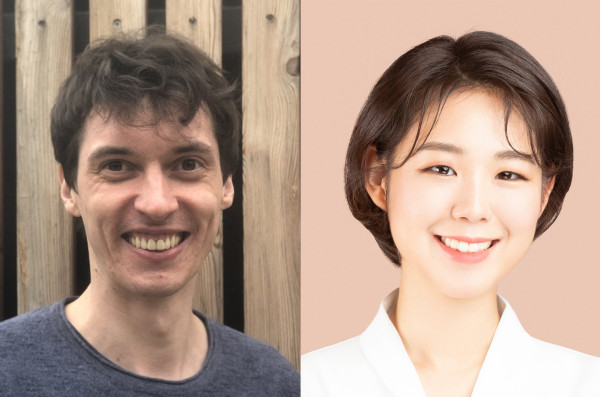
Each seminar consists of two presentations of 25 minutes each, allowing to present on a scientific question in depth, followed by time for discussion. The discussion is facilitated and timed by the chair.
Note that the format has been recently changed, as the pizzas will be served AFTER the seminars in order to facilitate discussions based on the talks just presented.
Onsite participation
12:15 — Seminars take place in EPFL room Coviz2 (MED 2 1124)
~13:15 — Pizzas will be served in the MED building atrium, second floor
Online participation
Starting at 12:15:
https://epfl.zoom.us/j/68368776745
Password: 1923
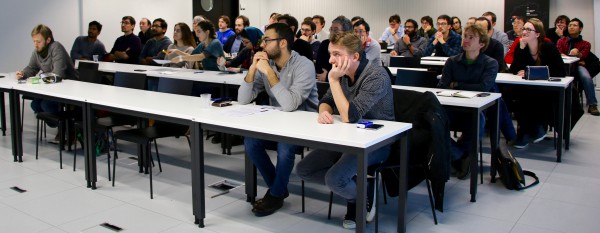
Abstracts
Talk 1 — Long-range descriptors in atomistic modeling beyond electrostatics
Philip Loche
Laboratory of computational science and modelling (COSMO), EPFL
Over the last decade, the use of machine learning based methods for modelling materials and molecules has developed rapidly. A key ingredient in most successful approaches has been the use of locality, also termed ”nearsightedness” of electronic models. Local models truncate atomic interaction beyond some cutoff radius which allows the development of fast algorithms scaling linearly with the number of atoms. What is systematically neglected in these models are any kind of long-range interactions interactions, including electrostatics, dipole-dipole or van der Waals forces, due to the relatively high computational cost involved
We present a general mathematical framework that is an extension of the long-range equivariant (LODE) descriptor, and can describe all long-rang interactions with an inverse power law form. It can be used to predict scalar target properties (energies) but can also be used in models that need to predict gradients of those (forces). We illustrate how this extension leads to significant improvements in both accuracy and computational cost.
Talk 2 — Automated crystallographic data interpretation and molecular property prediction for transition metal complexes
Yuri Cho
Computational molecular design laboratory (LCMD), EPFL
To exploit crystallographic repositories in data-driven applications for transition metal chemistry, one must determine the oxidation state and ground state spin of the metal centers from their crystal structures. For this task, we present cell2mol, a software that interprets crystallographic data of molecular crystals and retrieves the connectivity, the total charge of molecules, and the oxidation state of metal atoms. Furthermore, we present a general approach to predict the ground state spin of transition metal complexes by leveraging a database generated with cell2mol. We first curate a dataset of first-row transition metal complexes from the experimental crystal structures and their ground state spins from DFT computations. Then, using this dataset, we build empirical and statistical models that predict the ground state spin of transition metal complexes with a high accuracy of 97%. Lastly, we utilize our diverse dataset to benchmark the performance of various physics-based descriptors in predicting the molecular properties of transition metal complexes.
Check the list of the next MARVEL Junior Seminars here.
Low-volume newsletters, targeted to the scientific and industrial communities.
Subscribe to our newsletter