Sereina Riniker
Interview by Carey Sargent, EPFL, NCCR MARVEL, Mai 2020
The biggest challenge women scientists face is...
The most important is to look forward, to do our share and be role models for the next generation.
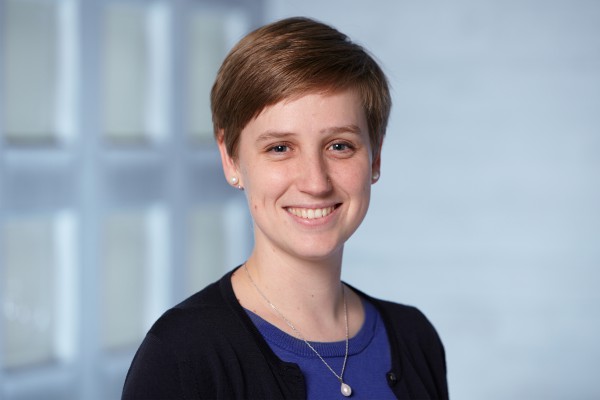
I chose a scientific career because…
I want to know how things work. I find it super fascinating how biological processes work in atomistic detail, how everything is connected. I like to understand mechanisms, not only in nature but also generally in engineering, society, etc.
If I weren’t a scientist, I would be…
I think I would have done something connected with arts. There are many similarities between arts and science, both are driven by curiosity and joy to experiment.
What is your greatest MARVEL discovery to date?
We have just joined MARVEL. I’m looking forward to exciting collaborations within this project.
My top two papers...
I like the papers where we presented a new idea or a proof of concept and thus these studies turned out to be stepping stones for whole research directions. In this respect, our paper on "replica-exchange enveloping distribution to estimate free-energy differences" for multiple states in a single simulation, and the one showcasing the idea of "molecular dynamics fingerprints as descriptors for machine learning" are some of my favorites.