Anna Paulish
Interview by Nicola Nosengo, NCCR MARVEL on 11 March 2024
Have you always been interested in science?
Mathematics and physics have always been my favorite subjects and I was very lucky with my teachers, who provided a great example and really sparked my interest. I also used to participate in competitions for young scientists back at school, and that was when I started to think that it would be great to continue doing this.
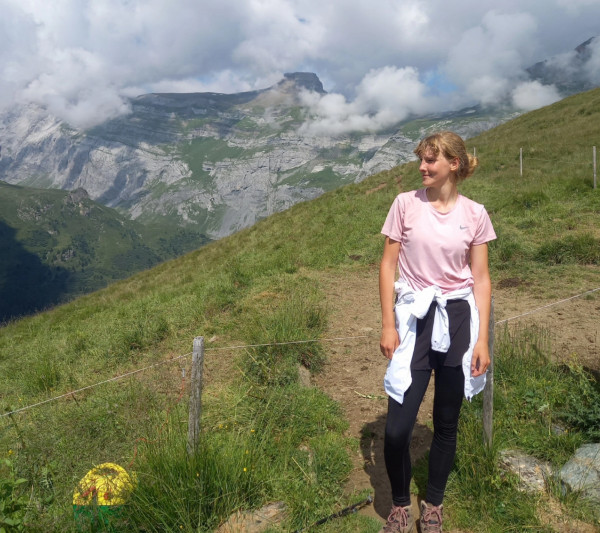
Anna Paulish
How did you hear about the INSPIRE Potentials Program?
I first heard that from my sister, who got this fellowship before - and my supervisor also told me about it and encouraged me to apply.
What is the topic of your master’s project?
The goal of the master’s project was to understand and explore generative modeling in applications to atomistic systems. The main focus of our work is on the application of generative modeling to glassy systems which are widely used materials in different applications. The typical procedure for modeling glassy material usually involves running the sequences of heating and cooling several times to get an example of the material that you need and each of them can be quite time consuming.
The relevant question then is: if we have several examples of amorphous structures, can we create new examples of any size by studying the statistical patterns observed within these systems? In my project, we present a neural network architecture designed for predicting atomic arrangements of local glassy environments by learning from examples generated using a machine-learning interaction potential trained on density functional theory calculations. We combine the well-known variational autoencoder with methods for generating symmetry-invariant representations of atomic arrangements. The focus was on applications to the generation of local motifs of amorphous silicon, as well as exploring a strategy for constructing entire structures.
Do you think women face specific challenges in the sciences?
Everyone sees that there are less girls studying and working as researchers in this field. For me, it’s very important when I see women at the higher stages of career. Having examples and role models, seeing they are human beings like you and that you can also try, really makes a difference.
Any advice for young girls interested in science?
I think it’s very important to look for opportunities and take advantage of them as they arise, maintaining curiosity in different areas. Explore them to find the topic you are truly interested in. This can be done by applying for internships, scholarships, or by using your free time and summer. There are many opportunities to gain experiences that can be helpful in choosing your future path.
What are your plans for the future?
I’m excited about the Ph.D. that I will start soon in the group of Prof. Michael Herbst, with extremely interesting research lines as well as many learning and professional growth opportunities. In general, I am interested in interdisciplinary research at the intersection of mathematics with materials science where I can apply the knowledge I gained through my studies to solve real-world problems. I believe that materials science plays a crucial role in shaping technological advancements and I am looking forward to contributing to bringing computational materials science to the next level.