Can quantum computers be the key to more natural and efficient modelling of materials?
by Nicola Nosengo, NCCR MARVEL
Many scientific disciplines are eager to exploit the power of quantum computing, once the technology is mature enough: medicine, climate science, cryptography, finance – the list could go on. But for computational material scientists, quantum computing holds a special promise. Current material modelling relies on methods such as density functional theory (DFT) to approximate the behavior of strongly correlated quantum systems (from atoms to molecules to complex crystal structures) so that they become tractable in the “classical” world of current computers. Using quantum computers to simulate the electronic structure of materials could greatly improve the accuracy, not only because quantum computers are largely thought to be more powerful than classical ones, but because their inner workings are much more similar to the very phenomenon that scientists are trying to reproduce. “As Richard Feynman put it, nature is not classical,” says Giuseppe Carleo, leader of the Computational Quantum Science lab at EPFL and a member of NCCR MARVEL since 2020. “If you want to simulate a quantum system, the best thing is to use another quantum system. In principle, with a quantum computer it should be possible to represent a many body wavefunction in a much more natural and more efficient way than with a classic computer”.
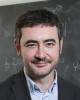
That is why, at the beginning of Phase III of NCCR Marvel, a new project dedicated to Leveraging Quantum Computers and Algorithms for Materials Discovery has been added to the research plan. Led by Carleo, the project involves Jürg Hutter from the University of Zurich, Ivano Tavernelli from IBM and Zoë Holmes from EPFL, who recently joined NCCR MARVEL.
Rather than looking towards a future of 100% quantum computing, the project aims to make the most of the current generation of hybrid quantum-classical computers. “It is the only reasonable paradigm we can use in the next few years” explains Carleo. “Current quantum computers do not allow us to work with more than 20 or 30 electrons, and the operations are noisy. We need to use them in conjunction with classical computing to make the system controllable”. This hybrid strategy has already proven promising, thanks to the availability of new hardware platforms. Take for example with the 2020 study by a group at Google that used a 12-qubit quantum system to simulate the binding energy of hydrogen chains and the isomerization mechanism of diazene. “Only a few years ago we would not have expected so many qubits to be available, and to be controlled,” says Carleo.
Still, there are many limitations in those platforms, and the main question behind the project is to what extent current hardware can be used efficiently and systematically for materials modelling.
One main goal of the project is to develop new quantum algorithms to be combined with more traditional ones that run on classical machines. To do this, the scientists will rely on quantum embedding, on which Jürg Hutter’s group in Zurich already has a strong experience. “The main idea of this method is to identify which electron orbitals are key in determining the properties of a material, and which ones are less active,” explains Carleo. “Identifying these active and non-active spaces is important also for classical calculations, but it is particularly crucial for quantum ones because we cannot afford to treat a large number of elements, given of how noisy these systems are. We want to make sure we concentrate our resources on the active spaces, the regions of the electrons that really matter”.
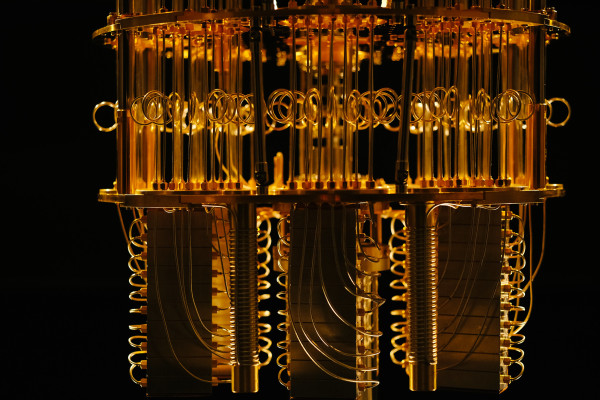
A IBM Quantum Dilution Refrigerator. Credit: Graham Carlow for IBM. CC BY-ND 2.0.
Machine learning also has an important role in the project. “In other parts of MARVEL it is used in a more data-driven way, for example to screen candidate materials, but we’ll use it in a slightly different way” says Carleo. “Our goal is to use neural networks to represent the many body wavefunction, on which we don’t have data a priori. So, in the classical part of the method we optimize the neural network so that it minimizes the energy and approaches the ground state as closely as possible. Then we try to improve the calculation using a quantum algorithm”. In the end, Carleo explains, the resulting state is the ground state calculated on the classical machine , times the wavefunction obtained on the quantum computer. “The classical part does the heavy lifting and brings you very close to the exact solution, and then you hope the quantum part gives you the extra step that you are missing to reach the final accuracy. Maybe eventually we will be able to remove the classical part entirely and only work with the quantum one. But this is not going to happen anytime soon.”
After validating the methods on very simplified electronic structures, in the final phase of the project the scientists hope to move to more realistic systems. These will include two-dimensional materials, for which a large database exists in MARVEL, metal-organic complexes which are notoriously difficult to simulate with pure DFT approaches, and metal-organic frameworks for photocatalytic applications.
As for hardware, the project relies on a partnership with IBM to remotely access their resources US-based quantum computation resources. “We don’t have any quantum computer available to us on an exclusive basis,” says Carleo. “Almost nobody has, apart from very large companies”. Although remote access is very efficient, it can be challenging because of the very way the hybrid algorithms work. “Because of the way we work, we often need feedback” says Carleo. “You run a classical operation, you send the result to the quantum device that gives an answer, and you use it to improve the original calculation, and so on. To do this without continuous and exclusive access to the hardware is not trivial”. The quantum computer typically has a long queue of operations, and a research group may have access to the machine only for a few minutes, and then a few more minutes in the next hour. That is why the other main goal of the project, beside new algorithms, is to improve the interoperability of the classical software tools used on classical systems, from DFT to machine learning frameworks, and IBM’s dedicated quantum computing interface.
Low-volume newsletters, targeted to the scientific and industrial communities.
Subscribe to our newsletter