How machine learning can help predict the spectral properties of materials
By Nicola Nosengo/NCCR MARVEL
Many techniques in computational materials science require scientists to identify the right set of parameters that capture the physics of the specific material they are studying. Calculating these parameters from scratch is sometimes possible but costs a lot of time and computational power. Consequently, scientists are always eager to find more efficient ways to estimate them without doing the full calculation.
This is the case for Koopmans functionals, a promising approach to expand the power of density-functional theory so that it can be used to predict the spectral properties of materials (such as what frequencies of light a material absorbs), and not just their ground state (such as the optimal positions of the atoms in that material). The accuracy of Koopmans functionals relies on finding the right “screening parameters” for the system one is studying. “You can interpret the screening parameters as the degree to which the rest of the electrons in a system react to the addition or removal of an electron” explains Edward Linscott, a postdoc at the Center for Scientific Computing, Theory and Data of the Paul Scherrer Institute, and member of MARVEL. The term ‘screening’ refers to the fact that the other electrons obscure — or in other words, screen — the addition of the new electron to someone who is watching the system from the outside. Linscott continues: “And this electronic screening — the process of adding or removing an electron from the system — is precisely the physical process that we are interested in when we talk about spectral properties. For example, in solar cells by shining light on a photovoltaic material we eject electrons from it and generate an electrical current”. Density-functional theory is very bad at describing processes such as these, andhe screening parameters tell us the degree to which a DFT approximation is failing us, and the strength of the correction we need to apply in order to correctly redeem the situation”, says Linscott.
The downside of Koopmans functionals calculations is that they take a lot longer than their DFT counterparts, mostly because of the cost of having to calculate the screening parameters . But a new paper in npj Computational Materials shows that even a simple machine learning model, trained with a modest amount of data, can significantly reduce the time needed for calculating screening parameters in the Koopmans algorithm. The article is by Yannick Schubert from the University of Zurich — who started the project as his master’s thesis — Sandra Luber (Yannick’s PhD supervisor at UZH), MARVEL’s director Nicola Marzari, and Linscott himself.
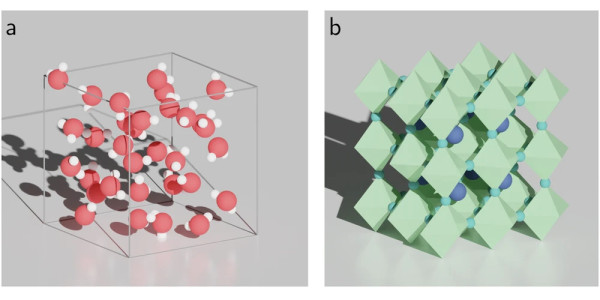
The two systems studied in this work. Liquid water, with oxygen atoms shown in red and hydrogen in white. b The halide perovskite CsSnI3, with caesium atoms in blue, tin polyhedra in green, and iodine in teal. From Schubert et al, npj Comput Mater (2024).
The authors chose two specific materials for their study: liquid water, and the halide perovskite CsSnI3. “They represent the kind of systems for which we thought we could make the most out of machine learning” explains Linscott. “Liquid water is naturally disordered and is out of the comfort zone for us scientists used to dealing with pristine crystals. Meanwhile, the halide perovskite is a promising material for use in solar cells, for which it is important to calculate how the changes with temperature”. To model the spectral properties of these systems with Koopmans functionals would require calculations on many copies of the same chemical system with different atomic positions. This process can be made much faster by training a machine learning model on a subset of these copies, and then using this model to predict the screening parameters for the remaining copies.
When the researchers set out, the question was: what kind of machine learning model would work, among the many options offered by the field? It turned out that a simple model, called ridge regression, would do. “We had a roadmap of increasingly sophisticated networks that we were going to try, and we did not know from the start if it was going to work or if we were going to be able to generate enough data” says Linscott. “To our surprise, the simplest model with very little data worked well. The model we ended up with is nothing like the sophisticated machine learning models that are all around us these days, but it was enough to accurately calculate screening parameters”.
While the network itself is simple, the scientists attribute this in part to the careful work they put into the construction of the “descriptors”, which are mathematical objects that must encapsulate the relevant physics of the system and are fed into the machine learning model.
While it would be possible to expand the method and make it more powerful with a more complex network and more training data, Linscott says that the next step will be to make the most of the method as it is now and use it to study the temperature-dependent spectral properties of interesting materials.
Reference
Schubert, Y., Luber, S., Marzari, N. et al. Predicting electronic screening for fast Koopmans spectral functional calculations. npj Comput Mater 10, 299 (2024). https://doi.org/10.1038/s41524-024-01484-3
Low-volume newsletters, targeted to the scientific and industrial communities.
Subscribe to our newsletter