Using alchemical compression and machine learning to describe high-entropy alloys
By Nicola Nosengo, NCCR MARVEL
High-entropy alloys, where 5 or more metals are mixed in roughly equal proportion, have been intriguing materials scientists for decades, since the seminal studies led by Jien-Wei Yeh and Brian Cantor in the early 2000s.
These experiments discovered that multi-element mixtures solidify in fewer different phases that one could expect given their composition, and in some cases even a single phase can be remarkably stable: a high-entropy alloy. Because they include so many elements, HEAs can display interesting behaviors for metallurgy and mechanics, and also for catalysis or water splitting. But modelling them computationally to predict how different metal mixtures may behave has proven elusive, and even current machine learning methods do not perform well beyond four or five elements.
To train the model, the researchers picked 25 elements from the d-block of the periodic table focusing on the transition metals that are most relevant for HEAs, and used them to generate 25,000 structures, each based on distorted bcc and fcc lattices, and including from 3 up to 25 randomly selected elements. They calculated the energy of each structure using Density Functional Theory (DFT). Then they let the model go through the set and learn correlations among the elements.
In particular, the model treats all metals in the set as if they were a combination of only three or four “pseudoelements”, and over several iterations learns to reproduce the DFT results by modelling those few fictitious pseudoelements, instead of treating each element as a completely distinct entity. This reduces the complexity and computational cost of calculations, and also leads the model to independently rediscover some of the similarities among elements that allow to group them in rows and columns along the periodic table. The model can also infer the behaviour of elements that were not in the training set, based on the degree of similarity with those that were included.
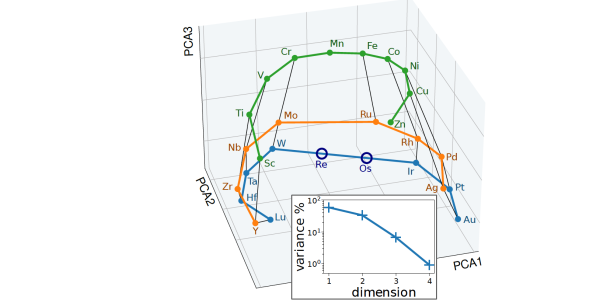
In an alchemical compression scheme, the elements are described as a combination of a small number of "pseudoelements". The figure represents the weights in this combination, and can be seen to reflect the arrangement of the transition metals in the periodic table.
“Technically the model is interpolating the missing elements based on those in the training set and using the alchemical compression as a blueprint to fill in the gaps”, notes Ceriotti. “But what it is doing in practice is more similar to an extrapolation, effectively predicting the behavior of elements it has never seen before. In a similar way, the model can guess interactions between elements that are not explicitly represented in the training set, reducing the data requirement and improving the transferability and stability of the model”.
After validating the model, the group used it for a few computational experiments. In the first one, they tried to simulate a mixture of all 25 elements, which resulted computationally in the formation of a disordered structure with strong element segregation. Some interesting data emerged – in particular, some metals show a tendency to associate and others tend to separate. These behaviors reflect the elements’ position in the periodic table, and also match some design rules already used by researchers working on HEAs.
Another experiment focused on the same alloy studied by Cantor’s seminal 2004 paper, CoCrFeMnNi. Starting from a homogeneous and disordered phase at high temperature, the experiment showed two phase transitions at 900 K and 400 K, characterized respectively by the ordering of chromium atoms and by the formation of nickel planes. The scientists also substituted manganese with molybdenum, which lead to similar, although less pronounced, ordering. Finally, they computed the noble metal alloy PdPtIrRuRh that shows a strong tendency to decompose into two distinct phases, PdPt and IrRhRu, indicating that the experimentally-observed high-entropy phase is likely to be kinetically stabilized.
Such observations can be used to define some data-driven rules for creating HEAs tailored to specific applications. Ceriotti says that his group keeps working with BASF to extend the framework, in particular to also include surfaces. One important application of HEAs could be in catalysis, and modelling surface behaviour is extremely important for it. “Catalizers based on high-entropy alloys would have several advantages”, Ceriotti explains. “Exactly because they have a high level of disorder, these alloys can better tolerate harsh reaction conditions. Additionally, they allow to obtain performances comparable to those of noble metals even with little or no actual noble-metal content”.
Reference
N. Lopanitsyna, G. Fraux, M. A. Springer, S. De, and M. Ceriotti, "Modeling high-entropy transition metal alloys with alchemical compression," Phys. Rev. Materials 7(4), 045802 (2023). DOI: 10.1103/PhysRevMaterials.7.045802
Low-volume newsletters, targeted to the scientific and industrial communities.
Subscribe to our newsletter