MARVEL research highlighted in JCP special issue on machine learning in chemical physics
by Carey Sargent, NCCR MARVEL, EPFL
The research paper of Alberto Fabrizio, a postdoc in Corminboeuf’s lab, and colleagues— Machine learning models of the energy curvature vs particle number for optimal tuning of long-range corrected functionals—was deemed an Editor’s Pick. The work examined how to use machine learning to help correct and make functionals used in density functional theory to describe the electronic structure of molecules more accurate.
Being able to describe the electronic structure of molecules, knowing how electrons are arranged in the most accurate way possible, sheds light on a molecule’s chemical properties. Density functional theory, a computational quantum mechanical modelling method, helps us investigate the electronic structure of many-body systems by using functionals of the spatially dependent electron density. It’s important to know however whether the functional being applied is reasonably accurate or not.
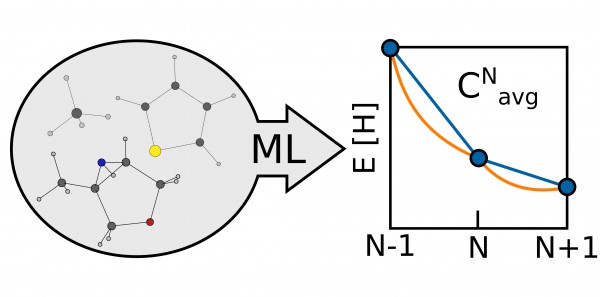
In the paper, the researchers identified a means of evaluating the quality of their approximations by looking at the average energy curvature as a function of the particle number, a molecule-specific quantity that measures the deviation of a given functional from the exact conditions of density functional theory. Since the energy of a molecule is linear as functional electrons are added, any deviation, or curvature, from this straight line reflects a difference between the functional and the true DFT value. Quantifying this information allows researchers to tune the functional and make it more accurate.
“This work was about finding a quick way to directly have an indicator of the quality of the approximation,” said Fabrizio. “That is, is the functional that I’m using okay or not?”
The technique of measuring the curvature to correct functionals had already been used, but, because of the computational cost, only with a handful of simple systems like hydrogen. In the paper, the researchers proposed a machine-learning framework that was able to target the average energy curvature between the neutral and the radical cation state of thousands of small organic molecules.
“The relation is something that was more or less known before in the sense that there has been much work in this field, but, finally, using machine learning and artificial intelligence gave us the possibility to treat thousands of molecules,” he said. “If you look at ten molecules you can start to draw a relationship or a kind of trend. If you have looked at 7000 molecules, it’s a bit more clear—you have a robust statistical relationship.”
Another interesting element of the work is that the researchers have gathered so much data that they can start investigating the relationship between the structure and composition of a molecule and this curvature. Using unsupervised learning techniques, they obtained information about the particular chemical patterns and molecular properties that may make a molecule problematic for DFT. Indeed, they have already learned that small, electron-dense molecules are very much exposed to this curvature problem.
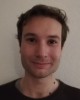
The work from Michele Ceriotti’s lab, Predicting molecular dipoles by combining atomic partial charges and atomic dipoles, presented a new framework for predicting gas-phase molecular dipole moments. Defined as the response of a molecule to an applied electric field, the molecular dipole is a central quantity in chemistry, a key element in the calculation of infrared and sum-frequency generation spectra, as well as in understanding intermolecular interactions.
Accurate calculations of the dipole moment, however, require time-consuming, high-level quantum mechanical calculations. In the paper, the researchers including Max Veit developed a ML method for predicting gas-phase dipole moments that achieves a high degree of transferability by combining an atomic charge - atomic dipole description that is rooted in physics with the conformational and chemical sensitivity afforded by kernel-based machine learning.
In order to improve the model performance, and to reveal the connection between an effective ML scheme and the underlying physical picture, they train three different models: one that incorporates local environment sensitivity into a simple scheme that predict scalar atomic partial charges, one that builds up the prediction as a sum of atom-centered , vectorial dipole moment predictions and, finally, a model that combines the two approaches. Using coupled-clusters reference calculations, they found accuracy comparable to that obtained by hybrid density functional theory.
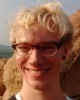
They then used a set of more complex compounds to push the models to their limits. The comparison between the performance of the different models allowed the researchers to discern the interplay of the different terms contributing to molecular polarization. The scalar model, for instance, is superior when describing large molecules whose dipole is almost entirely generated by charge separation. The combined model usually yields the best all-around performance, but the best combination of the scalar and vectorial contributions depend on the specifics of the system being examined. This finding indicates how important it is to account for both the local and non-local effects that contribute to the molecular dipole moment. Future models, particularly those developed to describe condensed phases, will pose additional challenges, in order to fully incorporate the long-range nature of dipolar interactions. The topic is the subject of another contribution of the Ceriotti group to this special issue, Incorporating long-range physics in atomic-scale machine learning.
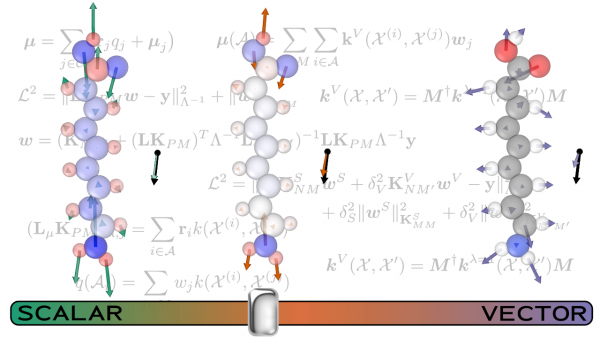
In the paper FCHL revisited: Faster and more accurate quantum machine learning, MARVEL postdoc Anders Christensen (from the lab of Anatole von Lilienfeld of the University of Basel) and colleagues presented a revision of their earlier representation of atomic environments in molecules or condensed-phase systems.
A number of data-driven machine learning models that can make predictions across chemical space with chemical accuracy have been introduced in recent years, and several machine learning models now provide the gradients necessary to perform tasks such as molecular dynamics simulations and geometry optimizations. The authors themselves previously published machine learning models based on the Faber–Christensen–Huang–Lilienfeld (FCHL18) representation, as well as a proof-of-concept implementation of learning and prediction of response properties such as atomic forces or dipole moments (necessary ingredients for the prediction of normal mode frequencies and IR spectra).
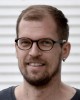
Though FCHL18-based models yielded state-of-the-art accuracy on several benchmark sets, its applicability was sometimes hindered by its computational cost, rendering comprehensive hyperparameter convergence prohibitive. While FCHL18 learns properties of chemical compounds by solving an analytical integral to compare atomic environments, other ML models use discretized representations, which can be handled with much greater computational efficiency.
In the paper, the authors presented such a discretized representation for chemical compounds based on a discretized version of FCHL18, dubbed FCHL19. They now fully optimized all model parameters to find a set of universally transferable hyperparameters that yields not only accurate but also computationally efficient ML models, without any further need for re-optimization. A review of different kernel-based force models from the literature that might be used with their representation has also been provided.
Benchmarking showed that ML models based on FCHL19 are able to yield predictions of atomic forces and energies of query compounds with chemical accuracy on the scale of milliseconds. The findings indicate that ML models with state-of-the-art accuracy can nowadays easily be used on hardware that is accessible to most chemists.
References:
Machine learning models of the energy curvature vs particle number for optimal tuning of long-range corrected functionals , Alberto Fabrizio, Benjamin Meyer, and Clemence Corminboeuf, J. Chem. Phys. 152, 154103 (2020); https://doi.org/10.1063/5.0005039
Predicting molecular dipole moments by combining atomic partial charges and atomic dipoles Max Veit, David M. Wilkins, Yang Yang, Robert A. DiStasio Jr., and Michele Ceriotti, J. Chem. Phys. 153, 024113 (2020); https://doi.org/10.1063/5.0009106
Incorporating long-range physics in atomic-scale machine learning, Andrea Grisafi and Michele Ceriotti, J. Chem. Phys. 151, 204105 (2019); https://doi.org/10.1063/1.5128375
FCHL revisited: Faster and more accurate quantum machine learning, Anders S. Christensen, Lars A. Bratholm, Felix A. Faber, and O. Anatole von Lilienfeld, J. Chem. Phys. 152, 044107 (2020); https://doi.org/10.1063/1.5126701
Low-volume newsletters, targeted to the scientific and industrial communities.
Subscribe to our newsletter