MARVEL labs collaborate to revolutionize computational metallurgy
by Carey Sargent, EPFL, NCCR MARVEL
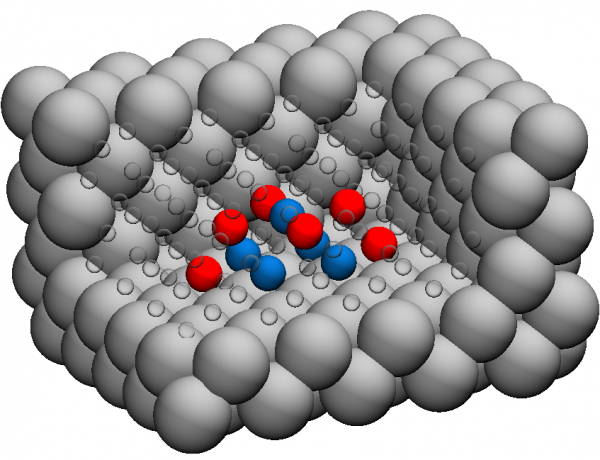
Snapshots of the simulation cells used to model single-formula-unit precipitates fully-encapsulated in the Al-matrix.
We’re at the beginning of what will turn out to be the way that computational metallurgy is done in the future.
— Bill Curtin, EPFL professor
Alloy properties such as strength and ductility ultimately depend on how atoms interact and form precipitates inside the base elemental metal. These precipitates inhibit the motion of dislocations – the carries of plasticity that make metals tough structural materials – and so strengthen the alloy. The combined expertise of EPFL professors Bill Curtin and Michele Ceriotti in mechanics of metals and machine learning, respectively, has allowed their groups to use artificial intelligence to generate powerful and accurate models for the interactions between atoms in the alloy that will lead to the ability to predict metallurgical properties.
The atomic interactions, called interatomic potentials, have traditionally been based on a general solid state physics understanding of how electrons respond when an atom is introduced into a metal. The resulting potentials have a few parameters to be determined, which is convenient when only a few experimentally-determined properties are available as yardsticks. The traditional potentials are however unable to deal with the physical situations that arise from the complex interactions in alloys with multiple atom types and complex crystal phases. In contrast, potentials generated by machine learning are rather built around a generic framework that can accommodate as much complexity as needed to describe all relevant atomic-scale properties of the material.
“I think a number of people in our field are just starting to realize that machine learning approaches are going to completely change how we develop interatomic potentials, particularly for very complex systems,” said Curtin, head of the Laboratory for Multiscale Mechanics Modeling at EPFL. “We’re at the beginning of what will turn out to be the way that computational metallurgy is done in the future.”
You are not at the level where some qualitative insight is enough to be relevant, you really have to be quantitative, and on problems that involve widely different time and length scales and in alloys that can easily contain ten elements, all of which are there for a reason.
— Michele Ceriotti, EPFL professor
These developments are coming at a critical time in the field. Metallurgists are not only looking for new alloys but are also striving to improve existing alloys including Aluminum, Magnesium, and Advanced Steels and adapt them to new additive manufacturing methods of production. For instance, the Al-6000 series of aluminum alloys contain varying percentages of magnesium and silicon and are commonly found in cars. Relatively lightweight, its strength can be greatly improved through engineered precipitation. Companies producing it need to understand how to control the processing, which reduces to controlling how atoms diffuse and react as a function of the time and temperature during the processing. Metallurgy has become so sophisticated that even the smallest details—a slight change in temperature capability– really matter and can make something worth doing economically…or not.
“From the point of view of modelling, this is the ultimate challenge,” said Ceriotti, head of the Laboratory of Computational Science and Modelling at EPFL. “You are not at the level where some qualitative insight is enough to be relevant, you really have to be quantitative, and on problems that involve widely different time and length scales and in alloys that can easily contain ten elements, all of which are there for a reason.”
The machine learning approach can accommodate this complexity because it is built on a set of training data supplied by high-quality first-principles electronic structure calculations that can be created for both existing and envisioned alloys. This training set, properly selected, teaches the model the correlations between structure and properties, and allows the scientists to harness the power of first-principles methods and get the accuracy of density functional theory (DFT) calculations at a lower computational cost. This approach allows researchers, in a brute-force sense, to machine-learn quantum mechanics for the particular material system of interest.
Curtin’s interest in the topic started with the visit of Prof. Ryo Kobayashi of the Nagoya Institute of Technology in Japan to his lab. During his stay, Kobayashi discussed his interest in neural networks, and Curtin saw how it could contribute to his work on the Al-6000 alloys.
“Once I could see that these methods allowed us to do things that we could just never do with traditional methods, I realized yes, this is the way things are going to go,” Curtin said. “We were largely at an impasse with existing approaches to potential development.”
One of the seminal papers to come out of the collaboration, “Neural network potential for Al-Mg-Si alloys,” was published in Physical Review Materials last year. In it, the authors showed how they created a neural network potential for the Al-Mg-Si alloy that has the accuracy to allow atomistic-level simulations of its processing and performance.
To do this, they used an extensive database of properties including the alloy’s complex precipitate phases to train the neural network for the Al-Mg-Si system. The generated potential accurately reproduced critical properties of pure Al relevant to mechanical behavior as well as heats of solution, solute-solute, solute-vacancy interaction energies, and formation energies of small solute clusters and complex precipitates all required for modeling the early stages of precipitation and mechanical strengthening. The work is the first neural network potential for complex alloys, demonstrating the power of this approach for metallurgy, and will enable Curtin and Ceriotti to perform detailed future studies of Al-Mg-Si.
While these first results on Al-Si-Mg are impressive, there is work to be done to streamline the design of ML models, and to make them robust enough to tackle problems of increasing complexity. Machine learning can, for example, be made more effective by providing a description of the atomic structures that encodes all of the fundamental symmetries of the laws of physics, Ceriotti said. The development of algorithms for machine-learning materials properties, as well as the statistical mechanics of materials, are the main interests of Ceriotti and his lab, COSMO.
The use of machine learning for materials in general, and alloys in particular, is still in its infancy. We want to push forward with applications to demonstrate what it can do, but also to develop tools that can be used by others to tackle different problems, and provide reference data that can be reused by the entire community. That’s how you get a bleeding-edge technology and turn it into the new industry standard.
— Michele Ceriotti, EPFL professor
As shown in the recent paper “Automatic Selection of Atomic Fingerprints and Reference Configurations for Machine-Learning Potentials,” letting a computer determine the most concise data representation is beneficial for both the accuracy and the computational efficiency of the machine-learning model—this model must also provide reliable estimates of the properties when encountering uncommon configurations, such as those that are observed during phase transitions or solid-state diffusion. Application of these advances has already led to an improved Al-Mg-Si potential that also captures key properties related to diffusion of atoms.
The thermodynamic description of the transitions between different phases of a material was the subject of another critical paper to come out of the collaboration, “Ab initio modelling of the early stages of precipitation in Al-6000 alloys,“ which was published in Acta Materialia, also last year. In it, the authors identified the chemical, surface, and elastic strain energies that contribute to the total precipitation energy versus size and composition. They further showed that overall trends using a DFT-based method are consistent with those generated using a model similar to the standard approach or classical nucleation theory. Finally, the predictions of the newest Al-Mg-Si machine learning potentials agree well with the first-principles results.
The results in these papers point toward the ultimate goal of the NCCR MARVEL collaboration, which is to link alloy-composition and processing to the final material properties for arbitrary alloy systems. To this end, the groups are focused on developing ML potentials as well as clear procedures for the computation of databases of properties so that they and other researchers can build potentials automatically.
“The use of machine learning for materials in general, and alloys in particular, is still in its infancy,” Ceriotti said. “We want to push forward with applications to demonstrate what it can do, but also to develop tools that can be used by others to tackle different problems, and provide reference data that can be reused by the entire community. That’s how you get a bleeding-edge technology and turn it into the new industry standard.”
Papers of interest
- Neural network potential for Al-Mg-Si alloys
- Ab initio modelling of the early stages of precipitation in Al-6000 alloys
- Automatic Selection of Atomic Fingerprints and Reference Configurations for Machine-Learning Potentials
- Hydrogen Diffusion and Trapping in α-Iron: The Role of Quantum and Anharmonic Fluctuations
Low-volume newsletters, targeted to the scientific and industrial communities.
Subscribe to our newsletter