New machine learning approach could accelerate materials optimization and drug discovery
Michele Ceriotti & Carey Sargent, EPFL, NCCR MARVEL
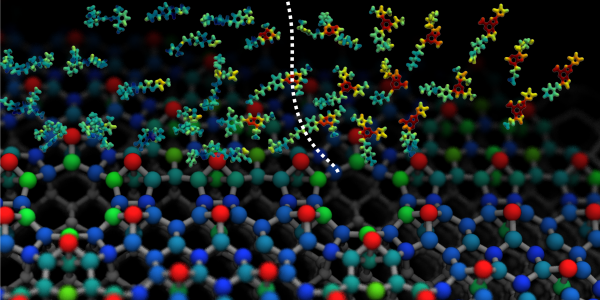
A machine learning algorithm can predict inexpensively the complex structure of silicon structures, and identify active (left) and inactive (right) drugs for a given target protein, using a small number of reference calculations or experiments.
Credits: Michele Ceriotti, EPFL and co-authors.
Researchers have designed an algorithm that uses just a few training references to predict whether or not a candidate drug molecule will bind to a target protein with 99% accuracy. This is equivalent to predicting with near-certainty the activity of hundreds of compounds after actually running only a couple dozen tests and could accelerate the screening of candidate molecules. The method is so precise that the single case in which it failed turned out to be due to a clerical error in the reference database.
The approach, developed by scientists from EPFL’s Laboratory of Computational Science and Modelling in collaboration with scientists at the University of Cambridge, the University of Warwick, the UK Science and Technology Facilities Council and the U.S. Naval Research Laboratory, can also identify which parts of the molecules are crucial for the interaction.
Researchers showed that the design of this algorithm, which combines local information from the neighborhood of each atom in a structure, makes it applicable across many different classes of chemical, materials science, and biochemical problems. The approach is remarkably successful in predicting the stability of organic molecules as well as the subtle properties of silicon surfaces that are crucial for microelectronic applications, and does so at a fraction of the computational effort involved in a quantum mechanical calculation.
The model at the heart of the machine-learning approach also provides insight into the range and energy scale of intermolecular forces and allows us to understand how various electronic-structure methods disagree in the description of different kinds of interactions. That is, machine learning not only changes the way we calculate the properties of materials and molecules, it also teaches us something about chemistry and materials science.
The research, which received funding from the NCCR MARVEL and the ERC Starting Grant HBMAP, illustrates how chemical and materials discovery is now benefitting from the Machine Learning and Artificial Intelligence approaches that already underlie disruptive technologies from self-driving cars to go-playing bots and automated medical diagnostics. New algorithms allow us to predict the behavior of new materials and molecules with great accuracy and little computational effort, saving time and money in the process.
Reference
Albert P. Bartók, Sandip De, Carl Poelking, Noam Bernstein, James R. Kermode, Gábor Csány, Michele Ceriotti, Machine learning unifies the modeling of materials and molecules, Science Advances 3, e1701816 (2017), doi: 10.1126/sciadv.1701816
Low-volume newsletters, targeted to the scientific and industrial communities.
Subscribe to our newsletter