MARVEL Junior Seminar — September 2020
Zoom meeting link:
https://epfl.zoom.us/j/93881551248
Password: 3417
The MARVEL Junior Seminars aim to intensify interactions between the MARVEL Junior scientists belonging to different research groups.
Each seminar consists of two presentations of 25 minutes each, allowing to present on a scientific question in depth, followed by time for discussion. The discussion is facilitated and timed by the chair.
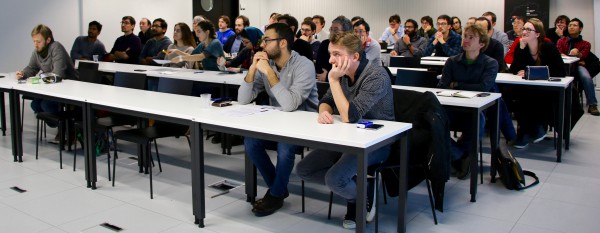
MARVEL Junior Seminar Organizing Committee — Michele Kotiuga, Sara Fiore, Stefano Falletta, Kristians Cernevics, Max Veit and Patrick Mayor
Talk 1 — Understanding structural diversity of metal-organic frameworks
Seyed Mohamad Moosavi1,2 , Aditya Nandy2 , Kevin M. Jablonka1 , Daniele Ongari1 , Heather J. Kulik2 and Berend Smit1
1Laboratory of Molecular Simulation (LSMO), EPFL, Sion
2Department of Chemical Engineering, Massachusetts Institute of Technology, Cambridge, USA
Millions of distinct metal-organic frameworks (MOFs) can be envisioned by combining metal nodes and organic linkers. This raises all sorts of questions related to how to explore this enormous chemical space to find the optimal material for an application. For example, at present, over 90,000 MOFs have been synthesized and over 500,000 predicted; how can we know if a new experimental or predicted structure adds new information. The chemical design space of MOFs can be seen as a combination of pore geometry, metal nodes, organic linkers, and functional groups. In this presentation, I will discuss our recent developments in machine learning representation of these domains, together with applying them to analyse the chemical diversity of MOF databases. This diversity analysis identifies biases in the current databases that has led to incorrect conclusions. The developed formalism to analyse diversity provides a simple and practical guideline to see whether new structures will have the potential for new insights, or constitute a relatively small variation of existing structures.
Talk 2 — An assessment of the fingerprints commonly used in machine learning
Behnam Parsaeifard1, Deb Sankar De1, Anders S. Christensen2, Felix A. Faber2, Anatole von Lilienfeld2, Emir Kocer3, Jörg Behler3, and Stefan Goedecker1
1Physics Department, University of Basel
2 Institute of Physical Chemistry, University of Basel
3 Institut für Physikalische Chemie, Goettingen University
Atomic environment fingerprints are widely used in computational materials science, from machine learning potentials to the quantification of similarities between atomic configurations. Many approaches to the construction of such fingerprints, also called structural descriptors, have been proposed. In this work, we compare the performance of fingerprints based on the Overlap Matrix(OM), the Smooth Overlap of Atomic Positions (SOAP), Behler-Parrinello atom-centered symmetry functions (ACSF), modified Behler-Parrinello symmetry functions (MBSF) used in the ANI-1ccxpotential and the Faber-Christensen-Huang-Lilienfeld (FCHL) fingerprint under various aspects. We study their ability to resolve differences in local environments and in particular examine whether there are certain atomic movements that leave the fingerprints exactly or nearly invariant. For this purpose, we introduce a sensitivity matrix whose eigenvalues quantify the effect of atomic displacement modes on the fingerprint. Further, we check whether these displacements correlate with the variation of localized physical quantities such as forces. Finally, we extend our examination to the correlation between molecular fingerprints obtained from the atomic fingerprints and global quantities of entire molecules.
Check the list of the next MARVEL Junior Seminars here.
Low-volume newsletters, targeted to the scientific and industrial communities.
Subscribe to our newsletter