MARVEL Junior Seminar — June 2019
The MARVEL Junior Seminars aim to intensify interactions between the MARVEL Junior scientists belonging to different research groups located at EPFL. The EPFL community interested in MARVEL research topics is very welcome to attend. We believe that these events will be central for establishing a vibrant community.
Each seminar consists of two presentations of 25 minutes each, allowing to present on a scientific question in depth, followed by time for discussion. The discussion is facilitated and timed by the chair.
Pizza is served at 11:45 in the MED hall (floor 0), and after the seminar at 13:30 you are cordially invited for coffee and dessert to continue discussion with the speakers.
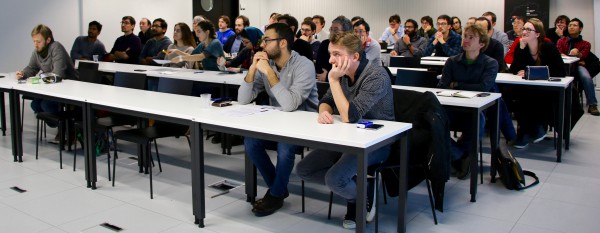
MARVEL Junior Seminar Organizing Committee — Davide Campi, Edgar Engel, Stefano Falletta, Michele Pizzochero, Kun-Han Lin, Francesco Maresca and Patrick Mayor
Abstract — Symmetry-adapted learning of tensorial properties in molecules and condensed-phases
Andrea Grisafi, David M. Wilkins, Michael J. Willatt, Michele Ceriotti
Laboratory of Computational Science and Modeling (COSMO), EPFL
Gaussian Process Regression (GPR) is a powerful method for nonlinear interpolation that makes use of a measurement of similarity between two systems.
In molecular physics and computational materials science, this method allows the prediction of atomistic properties like electronic energies and quantum forces, using the nuclear coordinates as the only input variables. These coordinates are used to construct the similarity functions (kernels) which enter the GPR framework. A great enhancement of the learning performances can be obtained when the kernel reflects the fundamental symmetries of the system at hand. For this reason, a great deal of effort has been invested in the past few years to construct kernel functions that are grounded in physical principles. In my presentation, I will introduce a symmetry-adapted extension of GPR that takes into account the symmetries of the O(3) group and that is suitable to interpolate any tensorial property of atomistic systems. This is made possible by decomposing the target tensor into spherical harmonic components and constructing covariant kernel functions of the proper angular momentum order. I will first show examples for dielectric response properties including molecular dipoles, polarizabilities and hyperpolarizabilities of water systems, as well as for the CCSD-level polarizability of a broad dataset of organic molecules. Finally, I will show that predicting the polarizability of a full molecular dynamics trajectory makes it possible to obtain anharmonic Raman spectra in the condensed phase.
Abstract — Learning (from) the electron density: transferability, conformational/chemical diversity
Alberto Fabrizio1, Andrea Grisafi2, Benjamin Meyer1, Michele Ceriotti2, Clémence Corminboeuf1
1 Laboratory for Computational Molecular Design, (LCMD), EPFL
2 Laboratory of Computational Science and Modeling (COSMO), EPFL
Intermolecular interactions are the cornerstone of chemistry beyond the single isolated molecule. Their undisputed importance is readily demonstrated by the intensive research effort spent achieving ever more accurate quantification of their magnitude and a chemically more intuitive characterization of their spatial behavior. Among all the molecular properties that encode the relevant information needed to fully characterize both intra- and intermolecular interactions, the molecular charge density occupies a preferential place due to its observable nature and its dependence only on three spatial variables. While, ρ(r) can be accurately obtained solving the electronic structure problem through ab-initio computations, this approach can become rapidly demanding if the electron density has to be evaluated for thousands of different molecules or for the very large chemical systems, such as peptides and proteins. To address this problem, we present a transferable and scalable machine-learning model of the electron density, able to predict the full density field directly from the atomic coordinates [1]. Additionally, we show how the regression model can be applied to access the information about intermolecular interactions in a chemically diverse ensemble of molecules derived from the BioFragment database (BFDb) [2]. Finally, we demonstrate the transferability of the model to new chemical situations, by predicting and analyzing the electron density field of a complex polypeptide.
[1] Grisafi, A.; Fabrizio, A.; Meyer, B.; Wilkins, D. M.; Corminboeuf, C.; Ceriotti, M. Transferable Machine-Learning Model of the Electron Density. ACS Cent. Sci. 2019, 5 (1), 57–64.
[2] Burns, L. A.; Faver, J. C.; Zheng, Z.; Marshall, M. S.; Smith, D. G. A.; Vanommeslaeghe, K.; MacKerell, A. D.; Merz, K. M.; Sherrill, C. D. The BioFragment Database (BFDb): An Open-Data Platform for Computational Chemistry Analysis of Noncovalent Interactions. J. Chem. Phys. 2017, 147 (16), 161727.
Check the list of the next MARVEL Junior Seminars here.
Low-volume newsletters, targeted to the scientific and industrial communities.
Subscribe to our newsletter